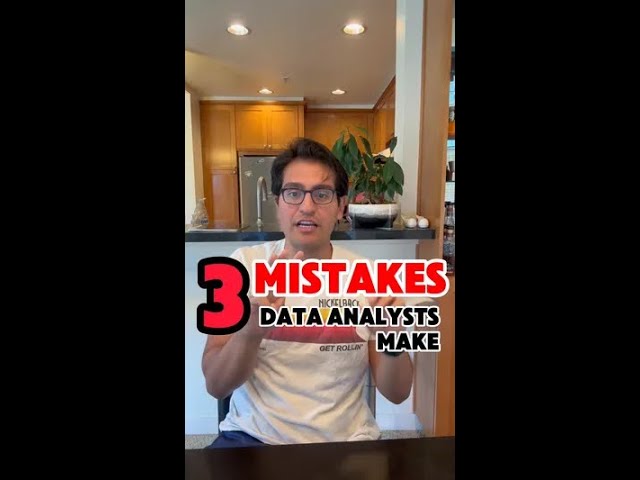
The 3 Biggest Mistakes Data Analysts Make and How to Fix Them
Data analysis is a powerful tool for driving business decisions, but even the most skilled analysts can fall into traps that hinder their effectiveness. In this article, we’ll explore the three most common mistakes that data analysts make, offering insights on how to avoid them and enhance your data-driven decision-making process.
1. Lack of Understanding of Business Context
One of the most critical errors data analysts make is not fully understanding the business context surrounding their work. Analysts often get engrossed in coding, building dashboards, or generating reports, sometimes losing sight of the bigger picture. This oversight can lead to misaligned efforts toward data exploration. Here’s how to remedy this mistake:
- Engage with business stakeholders: Regular interactions with business owners and stakeholders can clarify objectives.
- Define key goals: Clearly outline the goals of the data analysis before diving into execution. This ensures that analysts pull relevant insights and can actually drive business impact.
- Iterative feedback loops: Including continuous feedback from stakeholders throughout the process helps to keep the analysis aligned with business needs.
Impact: By grasping the business context, data analysts can produce more meaningful insights that lead to informed decisions, yielding measurable business outcomes.
2. Neglecting to Document Work
Documentation is a crucial component of data analysis that is often overlooked. Many analysts come from backgrounds that may not emphasize the importance of recording their processes, which can lead to significant issues down the line. Here’s why documentation is vital and how to ensure you do it:
- Types of documentation: Make sure to document code, frameworks, data architecture, and reasoning behind analytical choices. This aids others in understanding and building upon your work.
- Use version control: Platforms like GitHub can facilitate clear documentation of changes over time, helping to track the evolution of your analysis.
- Create a repository: Maintain a central repository for documentation and share it with your team to enhance collaboration and continuity.
Benefits: Well-documented work ensures that others can recreate or build upon your analysis, thus fostering collaboration and knowledge sharing within the team.
3. Ignoring Data Quality and Outliers
Ignoring data quality, especially related to outliers, is a significant mistake that can lead to faulty conclusions. Data analysts must be vigilant about the quality of the data they work with. Here’s how to effectively manage data quality:
- Recognize outliers: Understand that outliers can skew results. It’s essential to decide whether to include, exclude, or adjust these data points based on the analysis objectives.
- Implement data cleaning processes: Develop a systematic approach to cleaning data, which may involve methods like averaging, removing outliers, or using statistical techniques to account for them appropriately.
- Report on data quality: Always communicate about the data quality in your reports, informing stakeholders of any potential issues that may affect analysis outcomes.
Outcome: Prioritizing data quality leads to more reliable insights and better-informed decisions by stakeholders, which can significantly enhance the overall value of data analytics work.
Conclusion
Avoiding these three common mistakes can significantly improve the effectiveness of data analysis. By ensuring a clear understanding of business objectives, diligently documenting work, and maintaining a focus on data quality, analysts can create insights that genuinely drive business success.
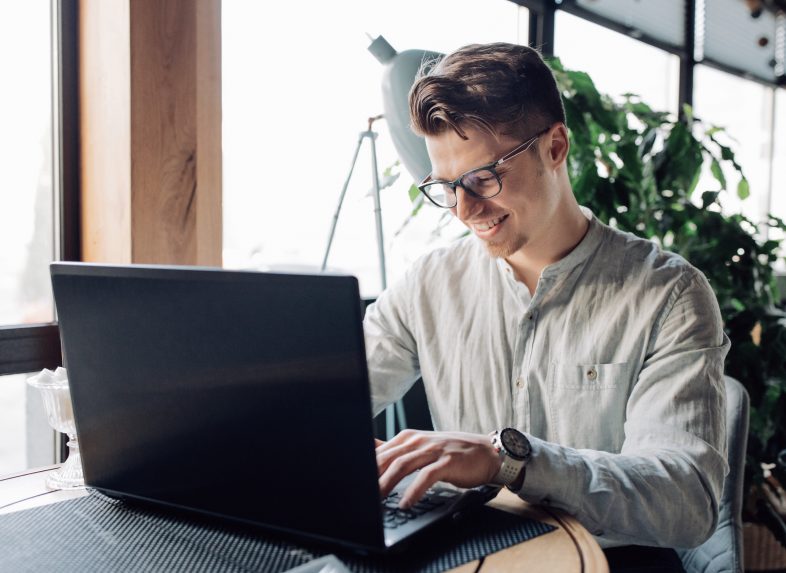
Unlock Your Career Potential
Frequently asked questions
Haven’t found what you’re looking for? Contact us at [email protected] — we’re here to help.
What is the Data Engineering Academy?
Data Engineering Academy is created by FAANG data engineers with decades of experience in hiring, managing, and training data engineers at FAANG companies. We know that it can be overwhelming to follow advice from reddit, google, or online certificates, so we’ve condensed everything that you need to learn data engineering while ALSO studying for the DE interview.
What is the curriculum like?
We understand technology is always changing, so learning the fundamentals is the way to go. You will have many interview questions in SQL, Python Algo and Python Dataframes (Pandas). From there, you will also have real life Data modeling and System Design questions. Finally, you will have real world AWS projects where you will get exposure to 30+ tools that are relevant to today’s industry. See here for further details on curriculum
How is DE Academy different from other courses?
DE Academy is not a traditional course, but rather emphasizes practical, hands-on learning experiences. The curriculum of DE Academy is developed in collaboration with industry experts and professionals. We know how to start your data engineering journey while ALSO studying for the job interview. We know it’s best to learn from real world projects that take weeks to complete instead of spending years with masters, certificates, etc.
Do you offer any 1-1 help?
Yes, we provide personal guidance, resume review, negotiation help and much more to go along with your data engineering training to get you to your next goal. If interested, reach out to [email protected]
Does Data Engineering Academy offer certification upon completion?
Yes! But only for our private clients and not for the digital package as our certificate holds value when companies see it on your resume.
What is the best way to learn data engineering?
The best way is to learn from the best data engineering courses while also studying for the data engineer interview.
Is it hard to become a data engineer?
Any transition in life has its challenges, but taking a data engineer online course is easier with the proper guidance from our FAANG coaches.
What are the job prospects for data engineers?
The data engineer job role is growing rapidly, as can be seen by google trends, with an entry level data engineer earning well over the 6-figure mark.
What are some common data engineer interview questions?
SQL and data modeling are the most common, but learning how to ace the SQL portion of the data engineer interview is just as important as learning SQL itself.