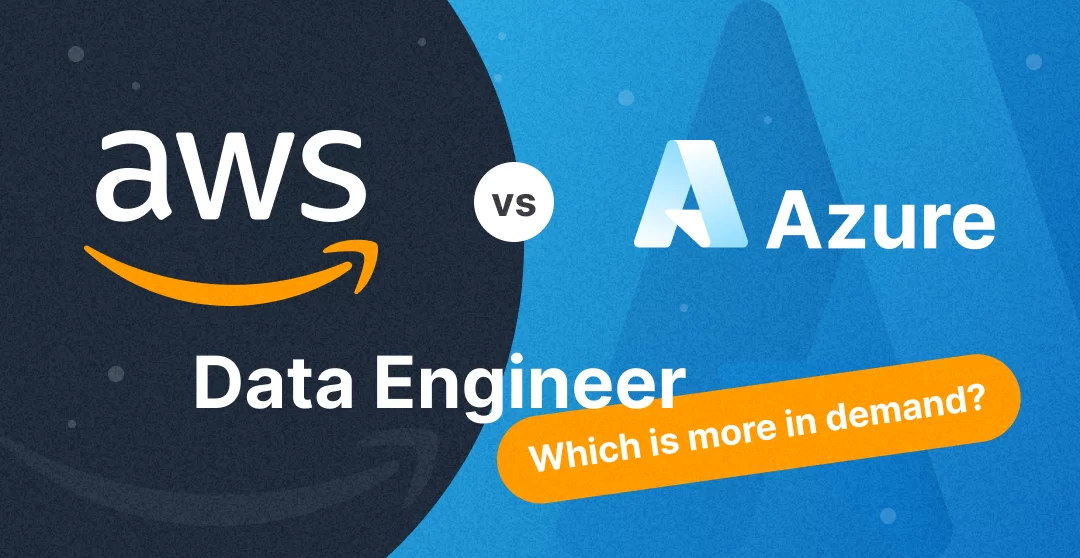
Aws vs Azure Data Engineering: Which is More in Demand?
As cloud technology increasingly underpins the technological frameworks of businesses globally, a critical question arises for both emerging and seasoned data engineers: Which platform, AWS or Azure, holds greater demand within the data engineering sector?
This article aims to dissect the current market dynamics concerning data engineering skills linked to AWS vs Azure. We will compare each platform’s unique strengths and offerings, examine the essential skills for mastering these environments, and align these with the industry’s current and forthcoming requirements. Additionally, we’ll scrutinize the insights and trends influencing data engineering’s future, thus offering a perspective on the shifting preferences within the employer landscape and the broader technology sector.
Overview of AWS and Azure in Data Engineering
AWS provides an extensive array of services that cater to the diverse needs of data engineers. Key offerings include Amazon S3 for data storage, Amazon Redshift for data warehousing, and AWS Glue for data integration and ETL processes. AWS’s strengths lie in its scalability, reliability, and comprehensive service offerings that cover virtually every aspect of data engineering. The platform’s maturity and wide adoption make it a standard in many sectors, equipping data engineers with tools to handle big data analytics, machine learning model deployment, and real-time data processing with ease.
Microsoft Azure, on the other hand, offers a compelling suite of services tailored for data engineering tasks. Azure Data Lake Storage for extensive data storage solutions, Azure Synapse Analytics for big data and data warehousing, and Azure Data Factory for data integration and ETL services are among its core offerings. Azure stands out for its seamless integration with Microsoft’s software ecosystem, including Power BI for analytics and SQL Server for database management. This integration makes Azure a preferred choice for enterprises entrenched in Microsoft’s ecosystem, offering robust solutions for data warehousing, big data analytics, and AI-driven data insights.
Recent statistics and market analyses reveal a growing demand for data engineers proficient in both AWS and Azure, reflecting the platforms’ critical role in the tech industry’s cloud-first strategy. Factors influencing this trend include the rapid digital transformation of businesses, the explosion of data generated by online activities, and the need for scalable, cloud-native data architectures. Industry adoption rates of AWS and Azure vary by sector and geography, with AWS traditionally leading in market share globally, but Azure gaining ground, especially among enterprises seeking deep integration with Microsoft products.
The demand for data engineers skilled in AWS and Azure is not uniform, with variations across industries such as finance, healthcare, and technology. In regions with a strong presence of technology startups, AWS skills may be more sought after, given AWS’s early dominance and extensive service offerings. Conversely, Azure sees higher demand in sectors with longstanding use of Microsoft solutions, benefiting from its integration with other Microsoft services.
Key Skills for AWS and Azure Data Engineers
Data engineering on AWS and Azure platforms requires a blend of foundational knowledge, technical skills, and platform-specific expertise. Mastery of these skills not only enhances employability but also drives career growth in the dynamic field of cloud data engineering.
Essential Skills and Certifications
- Cloud Architecture Understanding
Proficiency in designing scalable, reliable, and efficient data processing architectures using cloud services is fundamental. This includes knowledge of data storage options, compute services, and data orchestration tools offered by AWS and Azure.
- Data Storage and Database Management
Skills in managing relational and non-relational databases, such as AWS RDS, DynamoDB, Azure SQL Database, and Cosmos DB, are critical. Understanding how to design, implement, and maintain databases within these platforms is essential.
- Data Processing and Analytics
Experience with data processing tools (e.g., AWS Glue, Azure Data Factory) and analytics services (e.g., AWS Redshift, Azure Synapse Analytics) enables data engineers to build and optimize data pipelines for complex analytics workloads.
- Programming and Scripting
Proficiency in languages like Python, SQL, and Scala, and the ability to write, debug, and optimize code are key skills. These are used for data manipulation, ETL processes, and automation tasks.
- DevOps and Automation
Familiarity with CI/CD practices, infrastructure as code (IaC), and automation tools (e.g., AWS CloudFormation, Azure Resource Manager) helps in the efficient deployment and management of data infrastructure.
- Certifications
Pursuing platform-specific certifications, such as AWS Certified Data Analytics – Specialty or Azure Data Engineer Associate, validates expertise and enhances professional credibility.
Possessing a combination of these skills significantly boosts a data engineer’s employability across various industries that leverage cloud technologies. Certifications serve as a benchmark of competence, often leading to better job prospects, higher salaries, and roles with greater responsibility. As organizations increasingly adopt AWS and Azure for their data engineering needs, professionals skilled in these platforms are well-positioned for career advancement.
A comparative analysis of AWS and Azure reveals distinct strengths, weaknesses, and unique selling points for each platform from a data engineering perspective.
Feature | AWS | Azure |
Data Storage Options | Extensive, with services like S3 and Redshift | Broad, including Azure Blob Storage and Synapse Analytics |
Data Processing & Analytics | Mature offerings with EMR, Kinesis, and Glue | Comprehensive tools like HDInsight, Stream Analytics, and Data Factory |
Integration & Ecosystem | Strong integration within AWS services and third-party tools | Seamless integration with Microsoft products and a wide range of third-party services |
Learning Curve | Steep, due to the vast array of services and tools | Moderate, benefiting from Microsoft’s familiar interface and documentation |
Community & Support | Large community, extensive documentation, and resources | Growing community, with robust documentation and Microsoft support |
Strengths and Weaknesses
AWS: Strengths include a wider array of services and a more established presence in the market, offering greater flexibility and options for data engineers. However, its broad service catalog can introduce a steeper learning curve.
Azure: Strengths lie in its deep integration with other Microsoft products, making it an attractive option for organizations already invested in the Microsoft ecosystem. While Azure has made significant strides in data engineering capabilities, it is perceived to be playing catch-up with AWS in certain areas.
Real-World Use Cases of AWS and Azure in Data Engineering
Cloud skills are only as valuable as what you do with them. The way companies use AWS and Azure for data engineering shows how these tools stack up in the real world. Let’s look at clear examples of how top organizations put these platforms to work every day. This section covers hard-hitting use cases and tools that drive massive value in data projects, so you can see which skills pay off the most.
How Leading Companies Build Data Pipelines with AWS
Companies trust AWS to handle everything from raw data lakes to refined analytics. You’ll see it everywhere: retail giants, banks, streaming services — they’re all in on AWS. What’s the secret sauce? It’s a mix of scalable building blocks and deep integration.
Here’s what the flow often looks like:
- Amazon S3 stores raw and processed data. Walmart and Expedia both use S3 as their starting place — a centralized hub where all incoming data lands, whether from web clicks, IoT, or transaction records.
- AWS Glue automates the heavy lifting. Glue crawls data stored in S3, catalogs it, and runs ETL jobs. Let’s say a bank needs to clean up transaction logs every night. Glue takes care of that without manual coding, and business analysts get what they need the next morning — no long delays.
- Amazon Kinesis streams data at scale. Take Netflix as an example. Every time you hit play or pause, that event shoots through Kinesis. They process billions of these actions in real time, catching outages fast or recommending new shows with fresh data.
- Amazon Redshift powers analytics. Redshift is the end-point for reporting, machine learning models, and BI dashboards. Financial firms use Redshift to crunch market trends, run analytics, and serve results to traders within seconds.
A typical AWS stack allows teams to:
- Scale up or down instantly — no one wants to overpay for empty servers.
- Automate with serverless and managed tools, taking away hours of manual effort.
- Keep data secure with tight IAM controls, encryption, and audit logs.
It all comes together in a way that’s flexible and reliable, from first byte to last chart.
Azure Success Stories in Enterprise Data Workflows
Azure doesn’t just sit on the sidelines — it powers data workflows in global healthcare, finance, and manufacturing. The reason is simple: Azure brings together user-friendly data tools and tight business integration.
Here’s how it works for the big players:
- Azure Data Factory is the ETL backbone. Big insurance groups use Data Factory to connect legacy systems, cloud apps, and hundreds of data sources. Pipelines are built visually — think drag-and-drop, not code marathons.
- Azure Synapse Analytics ties it all together. A multinational retailer might use Synapse to collect sales data from every store, analyze it overnight, and feed custom dashboards by morning. Data flows from raw to curated to analyzed, all inside one workspace.
- Azure Databricks adds serious processing power. When global pharmaceutical companies need to analyze clinical trial results or genomics data, Databricks steps in. Spark clusters spin up — in minutes— and crunch huge datasets that would have stalled on old hardware.
- Seamless integration with existing Microsoft tools. Banks, manufacturers, and healthcare providers can hook up Office 365, Power BI, and SQL Server to their data pipelines. Reporting, alerts, and analysis fit into their everyday apps.
Enterprises pick Azure because:
- You get strong compliance and security controls out of the box.
- Legacy integration is easier, especially if the company already favors Microsoft tools.
- Rapid deployment lets teams try new workflows or scale up without major headaches.
Pulling it all together, real-world data engineering isn’t one-size-fits-all. Whether you pick AWS or Azure, you’ll find proven tools that help teams move faster and smarter. The question is — what do you want to build, and which stack fits best with your goals?
DE Academy Resources for Aspiring Data Engineers
At DE Academy, we offer meticulously crafted courses tailored to equip you with the skills to become a proficient data engineer in the cloud domain. Our offerings include specialized training in Amazon Web Services (AWS) and Microsoft Azure, two of the industry’s most prominent platforms.
AWS Data Engineering Courses
Our AWS course is designed to give you a comprehensive understanding of the most relevant AWS services for data engineering:
- Aurora-Athena using Glue: Dive into the integration of Amazon Aurora with Athena through AWS Glue to manage your data warehouse solutions effectively.
- AWS IAM Role: Learn to secure your AWS services and resources with Identity and Access Management (IAM) roles.
- AWS Glue Connection Crawler: Master the Glue Data Catalog to organize data discovery, simplify data search, and maintain metadata.
- Glue ETL Job: Gain hands-on experience in building and automating ETL jobs using AWS Glue to transform, clean, and enrich datasets.
- S3 to GCP Migration: Navigate through the complexities of cloud data migration from AWS S3 to Google Cloud Platform, and understand best practices for cross-cloud solutions.
- Cross-region S3-S3 migration using Lambda: Explore how to automate data transfer across different AWS regions using Lambda functions for optimized data management.
- DBT Postgres with Airflow – Windows: Implement DBT with Postgres databases and manage workflows with Apache Airflow on Windows environments.
Azure Data Engineering Courses
In our Azure-focused modules, you’ll learn to leverage Azure’s data engineering tools for robust ETL processes and analytics:
- ETL Pipeline – Azure ADF: Begin building ETL pipelines with Azure Data Factory (ADF), starting from resource setup to data loading and transformation.
Sing Up to learn ETL Pipeline Azure Documentation - Data Extraction: Get skilled in extracting data from various sources using Azure services.
- Raw_Hist_Layer: Understand the methods to process raw historical data layers within Azure’s architecture.
- Transformation_&_Loading: Learn the intricacies of data transformation and loading techniques specific to Azure environments.
- Azure_Resource_for_SCD1_Pipeline: Focus on implementing Slowly Changing Dimensions (SCD) Type 1 using Azure resources, a key concept for maintaining and updating warehouse data.
These courses are crafted to not just impart theoretical knowledge but to also provide hands-on, practical experience, ensuring that you’re job-ready for the demands of today’s data-driven organizations.
If you’re ready to advance your data engineering career, there’s no better time to start than now.
Integration with Machine Learning and AI: AWS vs Azure for Data Engineers
AWS offers a robust suite of machine learning and artificial intelligence AI tools tailored to meet the diverse needs of data engineers. Amazon SageMaker stands out as a comprehensive platform that simplifies building, training, and deploying ML models at scale. With built-in features such as Autopilot, which automates the creation of ML models, and Ground Truth, which helps generate high-quality labeled datasets, AWS empowers data engineers to integrate machine learning into their workflows seamlessly.
AWS also provides specialized AI services such as Amazon Rekognition for image and video analysis, Amazon Comprehend for natural language processing, and Amazon Forecast for time series predictions. These tools enable data engineers to leverage AI capabilities without needing deep ML expertise. Moreover, AWS offers deep integration with its data storage and processing services, such as S3, Redshift, and Glue, ensuring smooth data pipelines for machine learning tasks.
AWS’s focus on scalability and its wide array of APIs make it a preferred choice for large-scale AI data services. Data engineers can benefit from its AI/ML integration with big data processing tools like EMR (Elastic MapReduce), facilitating seamless handling of large datasets during ML model development.
Azure AI for data engineers
Azure offers an equally compelling ecosystem for AI and machine learning through its Azure Machine Learning platform. Designed with data engineers in mind, it provides a low-code and no-code interface for model training and deployment, alongside support for popular frameworks like TensorFlow, PyTorch, and Scikit-learn. This versatility makes Azure particularly attractive for organizations with varied data engineering requirements.
One of Azure’s unique strengths lies in its AI cognitive services, which include prebuilt APIs for tasks such as vision, speech, language, and decision-making. For example, Azure’s Text Analytics and Computer Vision services enable data engineers to integrate sophisticated AI functionalities into applications with minimal development overhead.
Azure also integrates tightly with its data services, such as Azure Synapse Analytics, Azure Data Factory, and Azure Databricks, providing a unified environment for end-to-end data and AI workflows. The integration of Azure AI with Power BI further enhances the ability to generate actionable insights from machine learning models, making it easier for data engineers to showcase results to stakeholders.
Key considerations for data engineers
- Both platforms support scalable machine learning solutions, but AWS’s emphasis on high-volume, global-scale deployments makes it ideal for projects with massive datasets.
- Azure’s user-friendly, low-code tools give it an edge for data engineers seeking quick deployment and iterative model development.
- AWS offers a broader set of specialized AI tools, while Azure excels in integrating AI with business intelligence and analytics.
In conclusion, AWS machine learning integration and Azure AI for data engineers each offer distinctive advantages. AWS is a powerhouse for data engineers aiming to build large-scale, flexible machine learning pipelines, while Azure provides a highly collaborative environment with tight-knit integrations for AI-driven data services. The choice ultimately depends on the specific use case, existing ecosystem, and skill set of the data engineering team.
Scalability and Performance: AWS vs Azure for Big Data Projects
AWS is renowned for its scalability and ability to handle massive big-data workloads. Its architecture is designed to automatically scale resources up or down based on demand, ensuring cost-efficiency and optimal performance. Services like Amazon EMR (Elastic MapReduce) and AWS Glue provide data engineers with the flexibility to process petabyte-scale datasets while benefiting from auto-scaling capabilities.
The Amazon Redshift data warehouse is a standout option for big data analytics, offering massive parallel processing and elastic scaling to accommodate fluctuating workloads. For real-time data processing, Amazon Kinesis enables seamless ingestion and analysis of streaming data, making it ideal for projects requiring high-speed performance.
In terms of performance, AWS’s global infrastructure ensures low latency and high availability, critical for big data projects with geographically distributed data sources. With tools like AWS Lambda, which enables serverless computing, data engineers can achieve highly responsive systems without managing underlying servers.
Azure scalability for big data projects
Azure also excels in scalability, particularly for organizations already invested in the Microsoft ecosystem. Azure Synapse Analytics (formerly Azure SQL Data Warehouse) is a robust solution for big data workloads, offering on-demand scalability and integration with other Azure services. For distributed data processing, Azure Databricks, built on Apache Spark, delivers high-performance analytics at scale.
Azure’s Event Hubs and Stream Analytics cater to real-time big data needs, allowing for scalable and efficient streaming data processing. Additionally, Azure Data Lake enables unlimited data storage and supports a variety of file formats, providing a flexible foundation for big data projects.
Azure’s global network of data centers and availability zones ensures reliable performance and minimal latency for geographically dispersed data. For dynamic workloads, Azure Virtual Machine Scale Sets and Azure Functions offer robust serverless and virtualized scaling options.
Performance comparison: AWS vs Azure
- Elasticity. Both AWS and Azure provide dynamic resource scaling, but AWS often edges out in terms of broader global infrastructure and diverse scaling options.
- Data processing speed. AWS’s Redshift and EMR are optimized for high-speed processing of large datasets, while Azure’s Synapse Analytics and Databricks focus on unified analytics and seamless data integration.
- Cost efficiency. AWS’s pricing model for on-demand scaling is competitive, but Azure’s tight integration with existing Microsoft tools can result in lower operational costs for enterprises using Microsoft software.
- Real-time performance. AWS Kinesis and Azure Event Hubs are comparable, but the choice may depend on specific project requirements and familiarity with the respective ecosystems.
Key Considerations for Big Data Projects
- Scalability. Both AWS and Azure are excellent for scaling big data workloads, but AWS’s global reach and auto-scaling features may better suit extremely large or globally distributed projects.
- Performance. AWS tends to lead in raw processing power, whereas Azure offers better integration for hybrid and Microsoft-centric environments.
- Ecosystem compatibility. For teams already utilizing Microsoft tools, Azure’s ecosystem provides seamless integration. For those seeking flexibility across various technologies, AWS is often preferred.
In summary, AWS scalability and big data with Azure offer robust solutions for handling large-scale projects. AWS is often the go-to choice for organizations prioritizing global infrastructure and high-speed performance, while Azure’s strengths lie in its integration with Microsoft services and hybrid cloud capabilities. Evaluating the specific needs of the project is essential to selecting the right platform.
Salary Comparison: AWS vs Azure Data Engineers
Wondering who brings home the bigger paycheck — AWS or Azure data engineers? Salary is often the first thing people want to know when picking between two cloud giants. There’s real money on the table, but things can shift based on skills, demand, and even your zip code. Let’s check the facts so you can chart your next move.
Global Salary Trends
When you look at the numbers, AWS data engineers usually have a slight lead in average pay. Employers tend to pay extra for AWS expertise, thanks to its longer foothold in the market and a steady wave of companies running on its cloud. But Azure is closing the gap fast, especially in large organizations tied into Microsoft products.
Recent studies show:
- Cloud data engineers (including AWS and Azure specialists) in the US earn a base average of $129,464 a year, according to Indeed’s reporting for 2025.
- AWS data engineers see typical salaries between $115,000 and $145,000 a year.
- Azure data engineers are commonly offered $110,000 to $135,000 a year.
The spread might seem narrow, but it adds up. Certifications, years of experience, and a knack for high-demand skills like real-time data streaming or big data analytics put your pay on the higher end, no matter the platform.
Job growth matters just as much as current pay. Projections through 2025 show that data engineer roles tied to cloud platforms — especially AWS and Azure — are set for double-digit growth worldwide. As companies race to the cloud, both skills stay hot.
If you want the details on how salaries break down for different data engineering roles, the latest Data Engineering Salary Guide 2025 gives a complete look, from ETL developers to cloud-focused engineers.
Regional Differences in Compensation
Where you work makes a huge difference. Some spots pay more for AWS, while others throw bigger offers at Azure pros. It’s all about local demand and what stacks companies use in your area.
Here’s what stands out:
- U.S. big cities — like San Francisco, Seattle, or New York — pay the most for AWS data engineering, thanks to heavy use in tech and finance. Azure can catch up fast in places where Microsoft partners are strong or where regulated industries (like healthcare and insurance) use Azure for compliance.
- Europe: London and Frankfurt offer high salaries for both AWS and Azure engineers, but local demand can tip the scales. UK job boards have reported a 10%–15% wage premium for AWS data roles, though Azure gains ground each year.
- India and Asia-Pacific: Azure is often favored by multinational firms with strong ties to Microsoft. Salaries are competitive, but AWS can command more in major tech hubs.
If you’re curious about how pay changes by city and country, or how market shifts affect Azure specialists in particular, check out this thorough breakdown for Azure Data Engineer salary potential. It covers how local demand, cost of living, and cloud adoption all play a part.
Here’s how location can shift data engineering pay between AWS and Azure:
- Urban tech centers. Expect AWS skill sets to get more attention and better pay.
- Government and enterprise-focused regions. Azure expertise moves higher, especially for public sector and highly regulated fields.
- Remote work. The surge in remote hiring can blur regional pay gaps, but top AWS and Azure engineers still find that company HQ location often sets base pay.
At the end of the day, both AWS and Azure data engineers take home strong salaries, no matter where you land. Focus on skill building and finding out what’s hot in your region.
Migrating Between AWS and Azure: Key Considerations
Swapping clouds isn’t just about copying files from Point A to Point B. When companies move workloads between AWS and Azure — or run both in a hybrid setup — everything from pipeline code to compliance rules gets tested. Getting it right matters for anyone in data engineering because the headaches (and big wins) happen behind the scenes. Let’s walk through what actually makes a migration tough and see which tools make your life easier.
Common Challenges During Migration
Switching platforms brings technical and operational snags you can’t ignore. These show up in both small and big projects, and they hit data engineering teams hard when shifting between AWS and Azure.
- Service mapping. AWS and Azure offer similar services (think S3 vs. Azure Blob Storage, or Redshift vs. Synapse Analytics). Trouble hits when features don’t match one-for-one. For example, automated ETL in AWS Glue might not have a perfect twin in Azure Data Factory, so pipelines need tweaks—or even a total rebuild.
- Data architecture differences. AWS and Azure have their own way of organizing networks, permissions, and security. IAM roles and policies in AWS work differently than Azure’s Active Directory and RBAC. Transferring those rules is more than a copy-paste job.
- Compliance and security. Every move is scored on compliance. Both providers offer deep security features, but policy naming, audit logs, and encryption keys don’t look the same. Regulations (GDPR, HIPAA, etc.) can demand manual checks and changes as you shift workloads.
- Downtime and data consistency. Moving big datasets across clouds? There’s a risk of downtime, missing files, or out-of-sync data. Planning for minimal downtime and running parallel pipelines is a smart move, but it adds work during the transition.
- Hidden costs. Moving data isn’t free. Cloud transfer fees, temporary resource spikes, and duplicated storage can balloon budgets if you’re not watching closely.
Tools to Simplify Cross-Cloud Transitions
Here’s the good part: you’re not alone on migration day. Both AWS and Azure offer migration suites, and there are solid third-party options made for data engineering moves. Picking the right tool can save weeks — or months — of manual work.
Some standout options include:
- AWS data migration service (DMS). Handles everything from simple file transfers to complex database moves. DMS supports cross-cloud transfers, so you can move from AWS to Azure SQL Database or vice versa. It even keeps data flowing during cutover, so you don’t fall out of sync.
- Azure migrate. Azure’s answer for smooth cloud moves. You get assessment tools, dependency mapping, and one-click moves for VMs, databases, and web apps. It’s handy for bulk shifts or when converting legacy infrastructure.
- CloudEndure (by AWS). Automates server migrations and disaster recovery across clouds. Works for both AWS and Azure targets.
- Third-party champions. Tools like Striim or Talend allow live data replication and pipeline migration across platforms. They’re great when you want abstraction and peace of mind, making hybrid and multi-cloud setups less painful.
- Terraform and infrastructure as code. For devs who want precision, tools like Terraform let you declare cloud resources in code and spin them up on AWS or Azure with small tweaks. This cuts out manual setup and avoids drift.
Pre-migration, audit your architecture. Use both clouds’ assessment tools to sniff out compatibility issues and cost surprises before flipping the switch.
Learning the tools and prepping for common sticking points means fewer surprises when it’s time to go hybrid or make a move. That’s the kind of data engineering skill that pays off again and again—especially when every migration has its own quirks.
Sign up at DE Academy and begin your learning journey with our AWS and Azure data engineering courses. Whether you are starting from scratch or looking to expand your expertise, our courses are designed to provide you with a deep understanding and practical experience in the most in-demand cloud services in the industry. Don’t miss this opportunity to elevate your skills and become a sought-after data engineering professional.