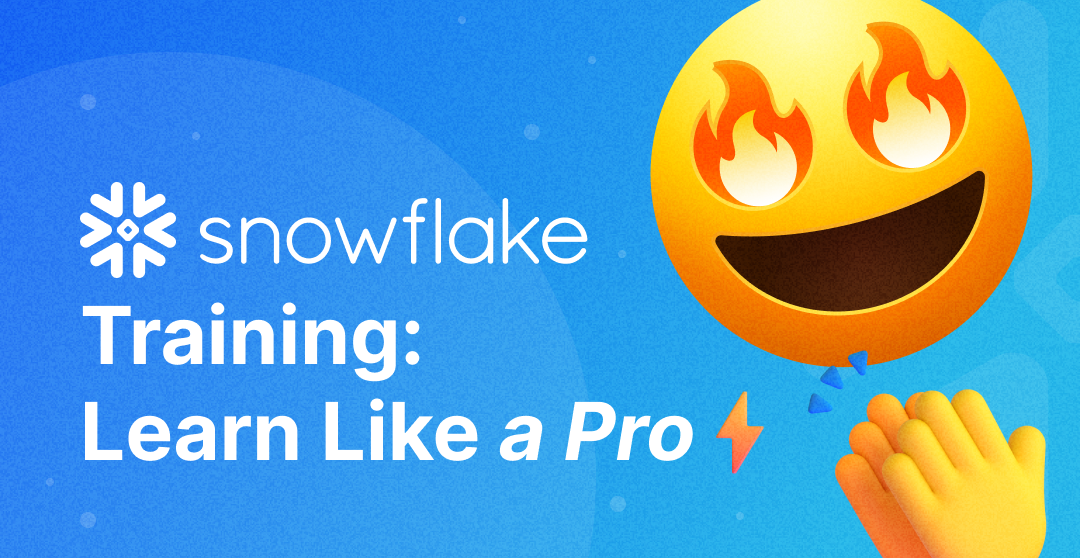
Snowflake Training: Learn Like a Pro
Data engineering is undergoing a major transformation. Traditional data warehouses struggle with scalability, cost, and real-time processing. Snowflake has emerged as the leading cloud-native data platform, designed to handle the demands of modern enterprises.
Why Learn Snowflake?
Top companies — including Fortune 500 giants — are migrating to Snowflake for several key reasons:
- Serverless architecture
- Seamless scalability
- Cross-cloud compatibility
Unlike traditional databases, Snowflake separates computing and storage, allowing businesses to optimize costs while maintaining high performance. This on-demand elasticity makes it the preferred choice for real-time analytics, machine learning pipelines, and large-scale ETL processes.
Career benefits: high-demand skills for data engineers, analysts, and cloud architects
Mastering Snowflake is a strategic move for anyone looking to transition into Data Engineering or advance their career in cloud analytics. With Snowflake expertise, professionals can secure high-paying roles such as:
- Data Engineer
- Cloud Architect
- Business Intelligence Developer
Companies are actively seeking Snowflake-certified professionals to build scalable, efficient, and cost-effective data pipelines in the cloud.
Data Engineer Academy: your path to Snowflake mastery
To excel in this field, hands-on experience is essential. Data Engineer Academy provides structured Snowflake training covering real-world applications—from data warehousing fundamentals to building optimized ETL pipelines. It offers:
- Industry-relevant projects
- Expert-led courses
- Career support
These resources equip professionals with the skills needed to thrive in cloud data engineering.
What is Snowflake? Understanding the Cloud Data Revolution
For decades, organizations relied on on-premises data warehouses like Teradata, Oracle Exadata, and IBM Netezza to store and process large-scale data. While these systems were effective in their time, they introduced major operational bottlenecks such as hardware limitations, costly infrastructure maintenance, and rigid scaling models.
The modern data landscape demands real-time processing, multi-cloud interoperability, and elastic compute capabilities — challenges that legacy warehouses were never designed to handle. As a result, enterprises began migrating to cloud-native architectures, leading to the rise of platforms like Amazon Redshift, Google BigQuery, and Snowflake.
Unlike traditional systems, Snowflake offers a completely decoupled compute and storage model, making it an on-demand, pay-as-you-use solution that eliminates the need for provisioning and managing physical infrastructure. This architecture is a game-changer for organizations aiming to optimize performance while minimizing costs.
Snowflake’s architecture
Unlike traditional databases that operate on monolithic infrastructures, Snowflake was built for the cloud from the ground up. It offers a multi-cluster shared data architecture, leveraging the following key design principles:
1. Separation of compute and storage
- Traditional data warehouses tightly couple compute and storage, meaning performance and storage scalability are interdependent. This results in over-provisioning of resources and unnecessary costs.
- Snowflake completely decouples compute from storage, allowing each layer to scale independently. Businesses only pay for what they use, optimizing both performance and cost.
📌 Example: A business running daily batch ETL jobs can keep its storage active 24/7 while provisioning compute power only when needed—dramatically reducing infrastructure costs.
2. Multi-cloud and cross-cloud capabilities
- Unlike Amazon Redshift, which is exclusive to AWS, Snowflake operates seamlessly across AWS, Google Cloud, and Microsoft Azure. This enables businesses to run multi-cloud strategies, improve redundancy, and avoid vendor lock-in.
- Snowflake’s cross-cloud replication ensures high availability and disaster recovery while maintaining consistent query performance across different cloud providers.
📌 Example: A global enterprise can store customer data across AWS (North America), Azure (Europe), and Google Cloud (Asia), ensuring regulatory compliance while maintaining seamless access through a single Snowflake instance.
3. Instant Elastic Scalability
- Traditional data warehouses require manual tuning, indexing, and provisioning to handle workload spikes.
- Snowflake’s auto-scaling virtual warehouses adjust dynamically based on query complexity, ensuring consistent performance even under high workloads.
- Multi-cluster computing enables workloads to be distributed across multiple nodes, reducing query latency and avoiding resource contention.
📌 Example: During peak business hours, Snowflake can automatically scale up to accommodate real-time analytics queries, then scale down during off-peak times to reduce costs.
Technical advantages of Snowflake over traditional warehouses:
Feature | Traditional warehouses (e.g., Teradata, Exadata) | Snowflake |
Compute & storage | Tightly coupled, requiring pre-provisioning | Fully decoupled, scalable on demand |
Scaling | Manual, complex, and expensive | Auto-scaling, dynamic cluster management |
Multi-cloud support | No native support (vendor-locked) | Runs on AWS, Azure, and GCP seamlessly |
Concurrency | Limited — query competition leads to bottlenecks | Multi-cluster architecture eliminates concurrency issues |
Data sharing | Requires complex ETL processes | Secure, real-time data sharing without copying |
Security | On-prem-based security models | End-to-end encryption, RBAC, and compliance-ready |
Cost model | High upfront investment, CapEx-heavy | Pay-as-you-go model, optimized OpEx |
As enterprises rapidly migrate to Snowflake, the demand for skilled Snowflake Data Engineers, Cloud Architects, and BI Developers is growing exponentially.
- High-paying career opportunities: Snowflake expertise is a premium skill in the job market.
- Cross-cloud compatibility: Learning Snowflake means understanding AWS, Azure, and GCP cloud ecosystems.
- Automation & scalability: Mastering Snowflake prepares engineers for large-scale data automation and analytics.
Job Market Insight: LinkedIn and Indeed list thousands of job openings for Snowflake-certified professionals, with salaries ranging from $120,000 to $180,000+ per year for experienced engineers.
The shift toward cloud-based, scalable data solutions is inevitable, and Snowflake is the platform that’s defining the future of data engineering. With its elastic architecture, seamless cloud integration, and cost-efficient model, Snowflake is a game-changer for businesses and a must-learn technology for data professionals.
For engineers looking to future-proof their careers, mastering Snowflake and cloud data engineering is one of the best career moves you can make today.
Snowflake in the Job Market: Why It’s a Must-Know Skill
Snowflake expertise is in high demand, especially in data engineering, analytics, cloud, and business intelligence roles. As organizations migrate to cloud data platforms, job postings requiring Snowflake skills have surged. In the U.S. alone, Indeed lists over 5,000 openings that mention “Snowflake,” spanning titles like Data Engineer, Snowflake Developer, and Cloud Architect. LinkedIn’s Emerging Jobs Report noted a 35% growth in data engineering jobs from 2015–2020, and Snowflake’s popularity is a key contributor to this trend. Roles frequently seeking Snowflake proficiency include Data Engineers, Data Architects, BI Analysts, and Data Scientists, reflecting how integral Snowflake has become in modern data stacks.
Industries adopting Snowflake are broad-based. Snowflake’s customer base spans finance, healthcare, retail, tech, and more.
This means sectors like financial services, healthcare, retail, and software are actively hiring Snowflake-skilled professionals. For instance, an analysis of Snowflake’s users shows top industries include IT services (21%), software (13%), and financial services (6%) of Snowflake’s customer share. Major companies have adopted Snowflake at scale – Fortune 500 giants such as Amazon, Walmart, ExxonMobil, Apple, CVS Health, and UnitedHealth Group all leverage Snowflake’s platform for their data needs. These adoptions drive demand for engineers who can design and manage Snowflake data warehouses. Even amidst a cooling in general tech hiring, Snowflake-focused roles remain hot due to enterprises modernizing their data infrastructure. In the UK, for example, Snowflake Data Engineer postings have shown a rising salary trend (median up 29% YoY) despite fewer listings, indicating companies are willing to pay a premium for these skills.
Salary Insights by Region and Role
Snowflake Data Engineers earn competitive salaries globally, often exceeding those of traditional data engineers:
- United States: Data engineers with Snowflake skills average around $100,000 per year (base salary). This is in line with or slightly above general data engineering averages (around $110–125k in many surveys). Notably, entry-level Snowflake engineers command about $70k–$90k, which “often outpaces peers working with older on-premise technologies”. Mid-career professionals specializing in Snowflake typically earn $90k–$120k, and senior Snowflake architects/engineers can see $130k–$160k+ (especially in major tech hubs). This indicates a clear skill premium for Snowflake expertise. For example, one U.S. survey noted Snowflake-certified professionals earn roughly 65% more than their non-certified counterparts ( ~$195k vs. ~$118k on average ), underscoring how valuable Snowflake credentials are in the market.
- Europe (UK & EU): In the UK, the median salary for a Snowflake Data Engineer is £77,500 per year, which is higher than the median for Data Engineers overall (around £70,000). This ~10% uplift suggests companies pay extra for Snowflake specialization. Top-quartile Snowflake roles in the UK offer £100k+ packages. Elsewhere in Europe, data engineering salaries vary – for example, general Data Engineers average around €70k in Germany and €50–60k in countries like Spain – and Snowflake-focused roles tend to sit at the higher end of these ranges. Snowflake’s demand in Europe is strong in finance and telco sectors adopting cloud data warehousing, which pushes salaries up.
- Asia (India & APAC): Snowflake talent is also well-compensated relative to local norms. In India, mid-level Snowflake Data Engineers (about 2–9 years experience) earn roughly ₹4–22 lakhs per annum (LPA), indicating mid-range salaries often in the teens LPA (₹10–15L or higher for senior-level in that band). This range is above many traditional BI/DBA roles. In fact, one analysis found that employees “who know Snowflake earn an average of ₹29.6 lakhs”, with the bulk in ₹19L–₹30L range and top experts earning well above ₹50L. Other Asia-Pacific markets like Singapore and Australia also see a premium – Snowflake data engineers in Singapore, for example, often earn salaries on par with global rates given the demand for cloud data skills in the region’s financial and tech companies. Overall, Snowflake specialization tends to boost compensation by 10–20% (or more with certification) compared to similar roles without Snowflake, across regions.
Snowflake vs. Traditional Data Engineer Pay: Employers value Snowflake proficiency as a specialized skill. In concrete terms, a Snowflake-focused engineer often earns more than a “traditional” data engineer (one working on legacy on-prem databases or older ETL tools). The UK data shows Snowflake engineers making ~£7K more than average data engineers. In the U.S., entry-level Snowflake roles start higher than many entry data engineering jobs in legacy environments.
At senior levels, Snowflake architects can command a significant premium, especially if they hold Snowflake’s SnowPro certifications. According to one report, Snowflake-certified pros in the US average ~$195K, which highlights how moving into Snowflake-centric roles can accelerate earnings.
In summary, companies are willing to pay extra for Snowflake skills given the platform’s popularity – this includes higher base salaries, plus incentives like bonuses for cloud certifications or Snowflake project experience.
Salary ranges by experience (US)
Career stage | Typical salary (USD) | Example roles |
Entry-level | $70,000 – $90,000 | Junior Data Engineer, Data Analyst |
Mid-career | $90,000 – $120,000 | Data Engineer, BI Developer |
Senior/Architect | $130,000 – $160,000+ | Sr. Data Engineer, Snowflake Architect |
Experience and Certification Impact: Beyond regional differences, experience level and certifications dramatically affect Snowflake salaries. Entry-level engineers or analysts who upskill in Snowflake can quickly reach the higher end of junior pay scales. Mid-level professionals (e.g. 5-10 years experience in data roles) who gain Snowflake expertise often see a bump into the six-figure range in the US, or equivalent boosts elsewhere. Senior data engineers who become Snowflake architects or lead engineers not only take on strategic responsibilities but also enjoy salaries well into the top 10% of tech pay (e.g. $150K+ in US, >£100K in UK).
Adding a Snowflake SnowPro certification can further increase market value – certified Snowflake practitioners are preferred for high-paying roles and leadership positions, as indicated by their substantially higher average pay. In practical terms, a SnowPro Core or Advanced cert can be a career catalyst, often leading to more interviews and leverage for negotiating salary. Employers interpret certification as proof of up-to-date skills, which is especially important in a fast-evolving platform like Snowflake.
Career Roadmap: How to Become a Snowflake Data Engineer
Embarking on a career as a Snowflake Data Engineer requires mastering both fundamental data concepts and Snowflake’s unique platform features. Below is a structured, actionable roadmap that integrates each learning milestone with the Data Engineer Academy’s hands-on courses and personalized training, ensuring you gain practical skills and industry-aligned knowledge at every step.
Foundational Knowledge: Understanding SQL & Cloud Data Warehousing
Begin by strengthening your foundation in SQL and data warehousing principles, as these are critical for any Snowflake engineer. Snowflake is a SQL-driven cloud data warehouse, so become proficient in writing efficient SQL queries and understanding how to optimize them for analytics. Focus on concepts like filtering, joins, and aggregation, as well as performance tuning techniques (e.g. avoiding unnecessary subqueries or using proper indexing). Equally important is grasping data warehouse fundamentals: understanding the difference between OLTP and OLAP systems and why warehouses favor techniques like indexing, partitioning, and caching to speed up analytical queries. For example, OLAP queries benefit from these optimizations to retrieve large datasets quickly, whereas OLTP systems prioritize fast transactions and data integrity. Familiarize yourself with schema design (star vs. snowflake schema), as well as concepts like denormalization for read-heavy workloads.
Hands-on Practice: Reinforce these fundamentals with guided training. Enroll in Data Engineer Academy’s SQL , which covers SQL basics, and cloud data warehousing concepts, and provides exercises to practice real-world scenarios. Through this course, you’ll learn to write complex SQL queries and gain exposure to cloud data services, building a strong base for Snowflake-specific learning.
Mastering Snowflake’s Core Features & Architecture
Next, dive into Snowflake’s architecture and core features. Snowflake introduced a unique multi-cluster, shared-data architecture that separates storage from computing, enabling each to scale independently. Spend time learning how this works in practice. Understand that data is stored centrally in Snowflake (on cloud storage) and compute comes in the form of virtual warehouses – independent MPP compute clusters that execute your queries. Each virtual warehouse can be scaled up (bigger servers) or out (multiple clusters for concurrency) without affecting others, which is key to Snowflake’s elastic performance and workload isolation. Grasping these concepts will help you design solutions that take full advantage of Snowflake’s scalability.
Explore Snowflake’s multi-cluster warehouses, auto-suspend/resume, and how its compute–storage separation allows near-infinite concurrency. Learn about data sharing, one of Snowflake’s powerful features: Snowflake can share data across accounts in real time without copying data, thanks to its secure data-sharing technology.
This means producers can grant consumers access to live data securely, with no ETL or replication. Similarly, study zero-copy cloning, which lets you instantly clone databases/tables without duplicating the data – any clone just refers to the original data until changes are made
chaosgenius.io. This feature is invaluable for creating test environments or backups without extra storage costs. Snowflake Time Travel (covered later) works with cloning to recover historical data easily.
Building ETL Pipelines & Data Engineering Projects
With fundamentals and core features in hand, the next step is to build data pipelines and projects on Snowflake. As a Snowflake Data Engineer, you’ll be designing ETL/ELT processes to ingest and transform data. Start with Snowflake’s native data loading/integration tools. Learn to use Snowflake’s Snowpipe for real-time or continuous data ingestion. Snowpipe allows Snowflake to automatically consume data files as soon as they land in a stage, loading micro-batches of data in minutes rather than waiting for scheduled bulk loads.
Practice setting up a Snowpipe: define a stage (e.g. an S3 bucket), a pipe with a COPY INTO command, and trigger automated loads via cloud events or REST API. This will teach you how Snowflake handles streaming data ingestion, a common task in modern data engineering.
Snowflake’s ability to handle semi-structured data is another important area. Unlike traditional warehouses, Snowflake can natively store and query JSON, Avro, Parquet, and XML using its VARIANT data type and dot notation querying. Make sure to get hands-on with loading semi-structured data into Snowflake and using functions like FLATTEN
or OBJECT_CONSTRUCT
to parse and generate JSON. Snowflake’s support for diverse data formats (structured and semi-structured) means you can ingest data without heavy pre-processing, which is a huge advantage. For example, try loading a JSON file of event data into a Snowflake table and query it with SQL (extracting fields in SELECT statements). Understanding how to use Snowflake’s file format options and table stages for this will be valuable in real projects.
When describing your projects or experience, be specific. For example: “Implemented a Snowflake-based data warehouse for e-commerce data, integrating data from S3 via Snowpipe for near-real-time ingestion, and used dbt to transform 50M+ records daily into analytics tables, improving query performance by 40% through proper clustering.” This gives concrete context and impact. In Data Engineer Academy’s career development resources, they suggest highlighting how you leveraged Snowflake’s features like data sharing or semi-structured data handling.
Next, prepare for interviews and certification. Many Snowflake Data Engineer interviews will test your understanding of Snowflake’s architecture and your ability to apply it. Be ready for questions like “How is Snowflake different from a traditional database?” – you should be able to discuss the compute-storage separation, automatic scaling, and other unique aspects (e.g. “Snowflake is a cloud-native warehouse that separates storage and compute for independent scaling, and it can automatically scale out with multi-cluster warehouses, unlike traditional monolithic databases). Expect questions on performance tuning (“What are some ways to optimize a slow Snowflake query?”) where you can mention clustering keys, using the result cache, right-sizing warehouses, etc.
Finally, leverage 1-on-1 mentorship and career support to transition into the job. Data Engineer Academy’s personalized program shines here – they provide extensive career services to help you land your target role. This includes refining your resume and LinkedIn (they will ensure keywords and projects are presented correctly), conducting mock interviews (technical and behavioral), and even applying to jobs on your behalf. The Academy team will “apply for you” to relevant data engineering jobs, utilizing proprietary tactics to boost interview odds.
Why Choose Data Engineer Academy for Snowflake Training?
1. Personalized mentorship learning
Unlike self-paced courses, Data Engineer Academy offers one-on-one coaching from experienced Snowflake Data Engineers. You’ll follow a custom learning plan, ensuring you focus on real-world Snowflake skills that align with your career goals. With weekly mentor sessions and 24/7 Slack support, you’re never stuck learning alone.
2. Real-world projects & practical training
Theory isn’t enough to land a job. That’s why the Academy focuses on hands-on projects, where you’ll build end-to-end Snowflake data pipelines, work with AWS/GCP/Azure integrations, and optimize warehouse performance. These projects mirror real job tasks, giving you portfolio-ready experience that stands out to recruiters.
3. Job placement & career support
The Academy doesn’t just teach — you get full career support:
- Resume & LinkedIn optimization
- Unlimited mock interviews (technical + behavioral)
- Direct recruiter connections & job referrals
- “We Apply For You” service to maximize job opportunities
- Salary negotiation & post-hire support
Many graduates land six-figure Snowflake Data Engineer roles with this all-in-one training-to-job approach.
If you want hands-on Snowflake experience, expert mentorship, and full job placement support, Data Engineer Academy is the best choice. You’ll graduate job-ready, with real-world Snowflake expertise and a team guiding you until you land your dream role.
Ready to start? Join Data Engineer Academy today and launch your career as a Snowflake Data Engineer!