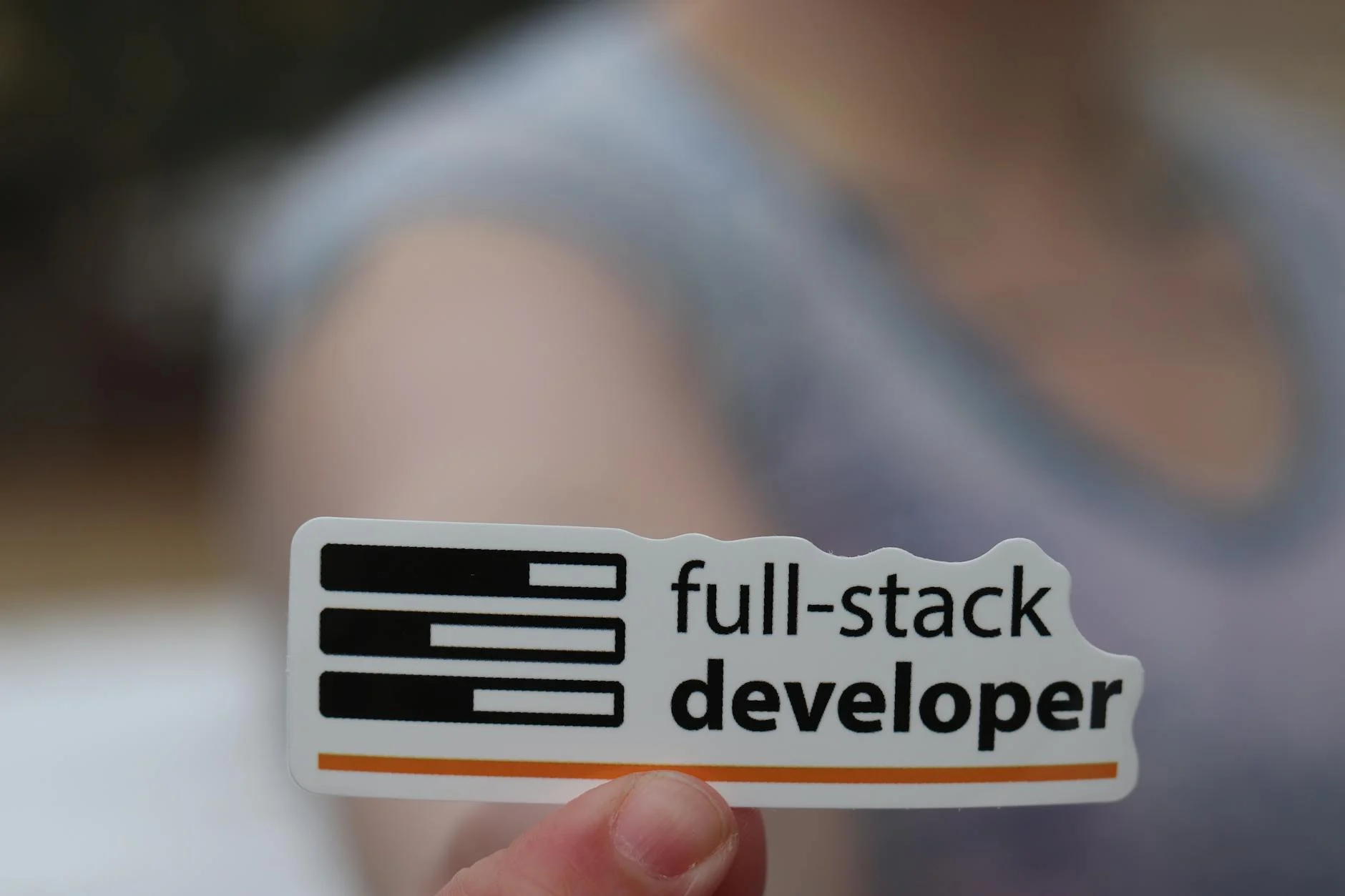
Why Companies Struggle to Hire Good Data Engineers
Finding and hiring good data engineers is tougher than you might think. Companies often struggle for a variety of reasons, ranging from unclear expectations to a disconnect between the skills they need and what candidates possess. This isn’t just a talent issue—it’s also about understanding the critical role data engineers play. These professionals are key in managing and processing vast amounts of data, yet many organizations undervalue that contribution, leading to low compensation offers and burnout among existing talent.
You might wonder why there’s such a gap in this crucial area. It boils down to a few key factors: a lack of formal education pathways for aspiring data engineers, an outdated view of the role, and inefficient hiring processes. If companies don’t recognize the skills and experience that really matter, they risk missing out on top talent.
In this post, we’ll break down the specific challenges companies face and discuss actionable insights to improve their hiring strategy. Whether you’re a system design learner, a data engineer, or considering a career change, understanding these dynamics can help you navigate this complex job market. For a deeper dive into the journey of becoming a data engineer, check out our Complete Guide: How to Become a Data Engineer in 2025.
The Growing Demand for Data Engineers
The landscape for data engineering is evolving rapidly, which brings along challenges and opportunities alike. As more companies recognize the importance of data in their decision-making processes, the demand for qualified data engineers has surged. This uptick not only sheds light on the necessity of skilled professionals but also highlights the complexities in filling these roles. Let’s explore the factors contributing to this growing demand.
Market Dynamics and Talent Scarcity
Competition for skilled data engineers is fierce. Companies are not just competing with each other; they are also contending with the ever-expanding technology sector, where data skills are becoming essential. The current job market reflects a high demand but a limited supply of qualified candidates, leading to what many describe as a talent crisis.
According to a report on data engineer job market trends, this growth is outpacing many other sectors, making data engineering one of the decade’s fastest-growing careers. Companies often resort to attractive compensation packages and perks to lure top talent, further complicating the situation. The scarcity of qualified data engineers means that firms need to reassess their hiring strategies and invest in developing talent from within to remain competitive.
Shifts in Job Requirements
Technology doesn’t sit still—and neither do the qualifications required for data engineering roles. As businesses begin to adopt new tools and methodologies, the skills needed by data engineers evolve. For instance, with the rise of artificial intelligence and machine learning, there’s now a heightened need for data engineers who can work with these technologies.
Job descriptions are changing, too. Today, data engineers need proficiency in tools such as cloud platforms (AWS, Azure), advanced programming languages (Python, Scala), and data warehousing solutions (Snowflake, BigQuery). The fundamental shift in “what” is required often leaves potential candidates feeling unprepared. Companies must recognize that this landscape is constantly changing and adapt their expectations and training programs accordingly.
Impact of Business Needs on Hiring Trends
Different industries have unique data needs, leading to diverse requirements for data engineering roles. For example, a healthcare company will seek data engineers who understand information security and compliance regulations, while an e-commerce business might prioritize data engineers adept at customer analytics and behavior modeling.
These specific demands create a landscape where companies struggle to find candidates whose skills align perfectly with their needs. Furthermore, firms focused on cloud technologies may prioritize engineers with experience in specific platforms. As a result, hiring managers should not only broaden their search horizons but also consider candidates with transferable skills, effectively widening the talent pool.
The journey for companies looking to navigate this competitive field can be daunting. However, understanding these dynamics problematizes the hiring process for data engineers and presents opportunities for strategic realignment. Whether you’re entering the field or navigating career advancement, staying informed about these trends is key to success.
For those looking to kickstart their journey, consider checking out the Key Concepts and Career Roadmap in 15 Minutes. Understanding the current landscape is the first step toward a successful career in data engineering.
Skill Gaps and Qualifications
When it comes to hiring data engineers, companies often overlook critical aspects that affect the talent pool. Understanding skill gaps and the qualifications valued in the hiring process is essential. This section focuses on two main areas: technical skills vs. soft skills and the significance of educational backgrounds and certifications.
Technical Skills Versus Soft Skills
In the competitive market of data engineering, the spotlight often shines brightly on technical skills. Proficiency in programming languages like Python, knowledge of tools such as Apache Spark, and experience with cloud platforms like AWS are all vital qualifications for a data engineer. However, the importance of soft skills should not be underestimated.
Soft skills, including communication, teamwork, and problem-solving, can be just as critical to success in this field. Data engineers often collaborate with data scientists, analysts, and other stakeholders to translate complex data requirements into actionable solutions.
Consider this: a technically skilled engineer may struggle to convey their findings to a non-technical audience effectively. This disconnect can lead to misinterpretation of data insights and hinder the decision-making process. Companies should look for a balance, assessing candidates for both technical abilities and interpersonal skills. After all, wouldn’t you prefer someone who can not only solve problems but also explain their solutions clearly? This approach leads to more well-rounded professionals who can thrive in a team-oriented environment.
Educational Backgrounds and Certifications
Now let’s explore the role of educational backgrounds and certifications in hiring. Traditionally, many companies have favored candidates with formal degrees in computer science or a related field. While a relevant degree often lays a solid foundation, this isn’t the only path to becoming a proficient data engineer.
More employers are recognizing the value of alternative educational routes, such as coding bootcamps or online courses, that focus on in-demand data engineering skills. This shift opens doors for diverse talent pools, allowing self-taught individuals to enter the field and fill prevalent skill gaps. If you’re curious about jumping into tech without a degree, you might find insights in our guide on How to get a tech job without a college degree.
Certifications, too, are gaining traction as a validation of one’s skills. Certifications from recognized platforms, such as AWS Certified Data Analytics or Google Professional Data Engineer, can enhance a candidate’s credibility in the eyes of employers. They not only demonstrate commitment but also a willingness to stay updated with industry standards and practices.
So, as hiring managers refine their processes, it’s crucial to appreciate both educational and experiential factors. By considering diverse backgrounds and recognizing the importance of ongoing education, companies can tap into a broader talent pool. This inclusive approach not only enriches the engineering team but also drives innovation within their organizations.
For more on the skill landscape, check out this article on how various companies are addressing the skills gap: The data skills gap keeps getting bigger. By staying informed and adaptable, companies can more effectively bridge the gaps in data engineering talent.
Recruitment Practices and Challenges
Navigating the hiring landscape for data engineers can be complex, and many companies inadvertently hinder their own recruitment efforts. Understanding recruitment practices and the challenges faced in this sector is crucial. Let’s break down a few significant issues affecting the hiring process.
Ineffective Job Descriptions
When it comes to attracting the right talent, clarity is key. Yet, many companies still write job descriptions that are either too vague or overly technical. A description lacking detail doesn’t just leave candidates guessing; it can turn them away entirely.
If a potential candidate can’t discern what the role truly entails, they’re likely to pass. Similarly, overly technical jargon can alienate strong applicants who may have the right skills but don’t speak the exact “language” used in the job ad. The key is to strike a balance—be specific about the required skills, but also explain the role in an approachable manner.
Consider this: A job post that not only lists technical skills like Python and SQL but also explains the day-to-day responsibilities, such as building data pipelines or collaborating with data scientists, is much more likely to resonate with the right candidates.
For insights on how to refine your job delineation, check out our post on How AI Transforms Data Engineering Hiring.
Lengthy Hiring Processes
Time is a luxury in the current job market. Lengthy hiring processes can lead to losing top talent, as candidates often have multiple offers to consider. Once they begin the job search, they expect a certain speed from prospective employers.
Consider this: If your hiring process drags on for weeks, candidates can grow disengaged and accept offers elsewhere. This situation often results from too many interviews or slow decision-making. It’s essential to streamline your process by reducing rounds of interviews and making quicker decisions based on clear evaluation criteria.
One way to enhance your hiring speed is by embracing technology, like AI in recruitment, to help screen and shortlist candidates faster. With the right tools, companies can better manage their timelines and avoid missing out on qualified data engineers. For more on tackling hiring challenges, refer to this article that discusses Challenges in Hiring Data Engineers.
The Role of Recruiters and Agencies
Recruiters play a vital role in connecting companies with the right candidates. However, they also face unique challenges, particularly when it comes to understanding what makes a strong data engineer. Many recruiters may excel in broader technical fields but struggle to pinpoint specialized skills essential for data roles.
Moreover, agencies may have to sift through an overwhelming volume of applicants. This process can lead to qualified candidates slipping through the cracks due to mismatched expectations or inadequate screening processes.
Recruiters can enhance their effectiveness by undergoing training to better understand the data engineering landscape. Having a clear grasp of key skills and current market trends can vastly improve the quality of candidate placements.
If you’re interested in improving your understanding of the data engineer interview process, consider reading Understanding the Data Engineer Interview Process.
In summary, addressing these recruitment practices and challenges will greatly improve your hiring strategy. By refining job descriptions, speeding up hiring processes, and empowering recruiters, companies can significantly improve their chances of attracting top data engineering talent.
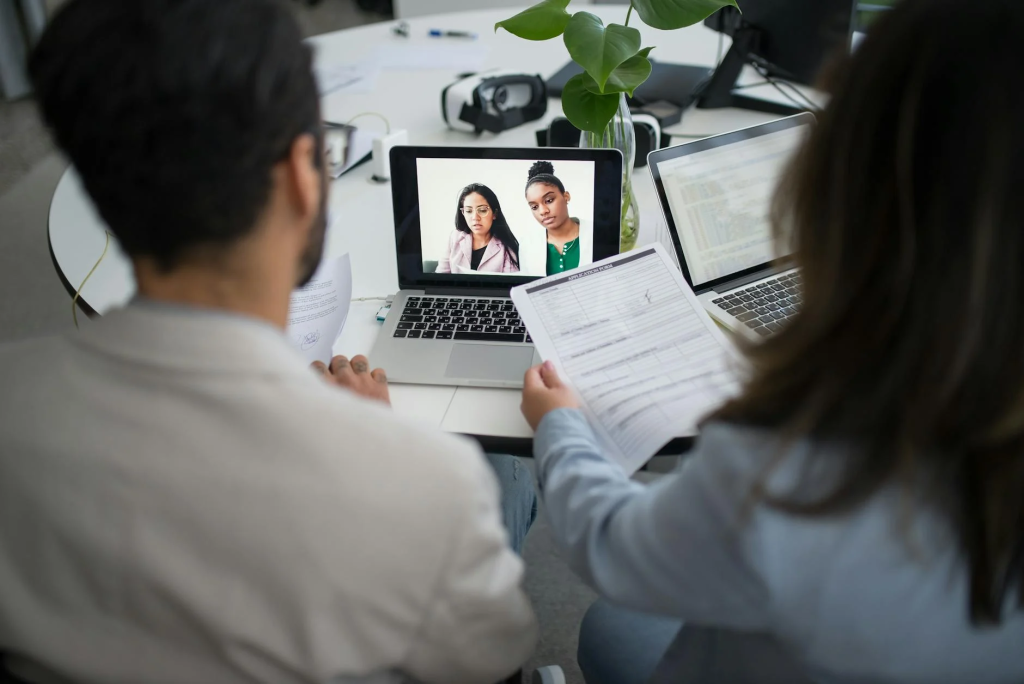
Emphasizing Skills Over Titles
Finally, as hiring managers and companies reassess what they’re looking for, it’s vital to emphasize relevant experience and skills over formal titles. The technical landscape is ever-changing, and many successful data engineers come from diverse educational backgrounds. Verifying candidates’ experience and their ability to adapt to new tools can open doors to exceptional talent that may otherwise be overlooked.
Consider revisiting candidates who may not perfectly match your initial criteria but bring a wealth of useful experience. This could lead to surprising results and impressive additions to your team.
By embracing these strategies, organizations can combat their hiring challenges and effectively bring high-quality data engineering talent into their fold. Whether through better assessment of needs, streamlined processes, or investing in company culture, the path to hiring success is clear. You have the power to transform how you attract future data engineers, paving the way for a more robust, data-informed organization.
Real stories of student success
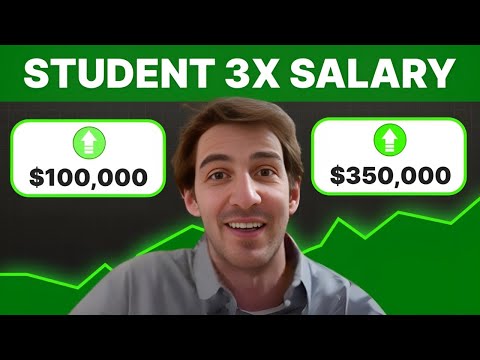
Student TRIPLES Salary with Data Engineer Academy
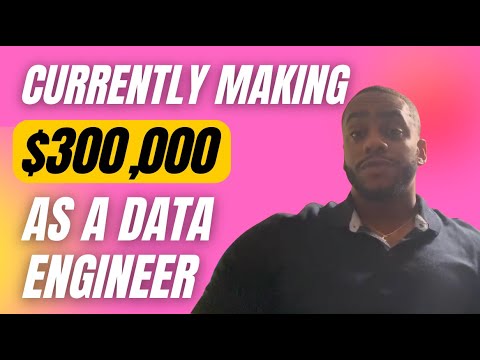
DEA Testimonial – A Client’s Success Story at Data Engineer Academy
Frequently asked questions
Haven’t found what you’re looking for? Contact us at [email protected] — we’re here to help.
What is the Data Engineering Academy?
Data Engineering Academy is created by FAANG data engineers with decades of experience in hiring, managing, and training data engineers at FAANG companies. We know that it can be overwhelming to follow advice from reddit, google, or online certificates, so we’ve condensed everything that you need to learn data engineering while ALSO studying for the DE interview.
What is the curriculum like?
We understand technology is always changing, so learning the fundamentals is the way to go. You will have many interview questions in SQL, Python Algo and Python Dataframes (Pandas). From there, you will also have real life Data modeling and System Design questions. Finally, you will have real world AWS projects where you will get exposure to 30+ tools that are relevant to today’s industry. See here for further details on curriculum
How is DE Academy different from other courses?
DE Academy is not a traditional course, but rather emphasizes practical, hands-on learning experiences. The curriculum of DE Academy is developed in collaboration with industry experts and professionals. We know how to start your data engineering journey while ALSO studying for the job interview. We know it’s best to learn from real world projects that take weeks to complete instead of spending years with masters, certificates, etc.
Do you offer any 1-1 help?
Yes, we provide personal guidance, resume review, negotiation help and much more to go along with your data engineering training to get you to your next goal. If interested, reach out to [email protected]
Does Data Engineering Academy offer certification upon completion?
Yes! But only for our private clients and not for the digital package as our certificate holds value when companies see it on your resume.
What is the best way to learn data engineering?
The best way is to learn from the best data engineering courses while also studying for the data engineer interview.
Is it hard to become a data engineer?
Any transition in life has its challenges, but taking a data engineer online course is easier with the proper guidance from our FAANG coaches.
What are the job prospects for data engineers?
The data engineer job role is growing rapidly, as can be seen by google trends, with an entry level data engineer earning well over the 6-figure mark.
What are some common data engineer interview questions?
SQL and data modeling are the most common, but learning how to ace the SQL portion of the data engineer interview is just as important as learning SQL itself.