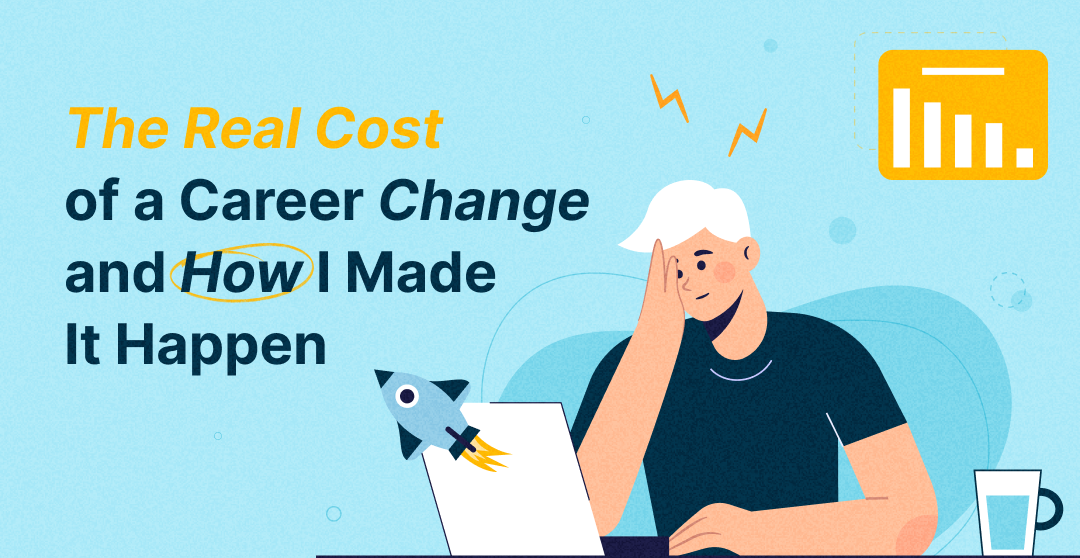
The Real Cost of a Career Change and How I Made It Happen
Switching to a data engineering career comes with more than just financial questions. It means real changes in your daily life, your mindset, and sometimes your bank account. Many career switchers face emotional stress and the pressure of stepping into a highly technical field. In the US, salaries for data engineers reflect why so many make the leap — entry-level roles start around $80,000–$110,000, and seasoned professionals can earn $130,000 or more.
But getting there isn’t free or easy. It often involves months of training, missed paychecks, and the challenge of learning entirely new systems. Nearly 40% of career changers say they switch for higher pay, yet about half of Americans still see education as a major barrier. Personalized training stands out as a proven way to bridge that gap, focusing on your background and goals to make the transition smoother and faster.
If you’re considering a switch or are already moving toward data engineering, this guide breaks down what you’ll actually face and how personalized support can set you up for real, lasting success. For more on salaries and the benefits of specialized skill development, see the full breakdown.
Understanding the Hidden and Obvious Costs of a Career Change to Data Engineering
Changing careers to data engineering requires more than just technical learning — it’s a commitment that comes with direct costs, personal sacrifices, and a change in mindset. Many underestimate these factors when starting out. This section details the price you pay — financially, emotionally, and through lifestyle shifts — so you know what to expect before making this move.
Education, Certifications, and Resources
Investing in education is the first big step. Tuition for specialized data engineering programs can range from a few hundred to several thousand dollars. Online course platforms can offer low-cost options, but deeper mentorship and job guarantees often require higher fees.
Primary expenses include:
- Tuition for bootcamps or mentorship programs, which often provide faster job placement than traditional degrees.
- Online course subscriptions and learning platforms.
- Books and technical manuals covering SQL, Python, cloud platforms, and system design.
- Required hardware upgrades—e.g., a modern laptop with enough RAM and processing power.
- Software costs, which can include licenses for data tools or cloud services.
People often save money by focusing on programs that structure learning around personalized guidance and industry needs. According to US data, top programs lead to starting salaries of $94k–$110k, with senior roles earning up to $200k or more. This makes the upfront cost justified for many, especially if you select paths offering job placement support. For a deeper breakdown of costs and what’s needed for a winning portfolio, refer to the skills required for data engineer roles.
Opportunity and Lifestyle Costs
Switching to data engineering often means adjusting your daily routine. Most career changers must find extra hours after work, sacrificing evenings and weekends to study. Many experience a temporary pay cut if they leave a job to focus on training or take internships to gain practical experience.
Common lifestyle costs include:
- Less free time, especially in the first few months. Many spend 30–60 minutes a day, but consistent effort pays off.
- Delayed social plans and missed family events.
- The real possibility of an initial salary drop, especially for those transitioning from senior roles in other industries.
- Emotional toll from juggling work, study, and personal responsibilities.
Real stories highlight people—from nurses and construction managers to finance professionals—who took on this challenge. Some started with no tech background and, with the right approach, landed roles in less than six months. Others who balanced part-time learning managed to upskill while keeping their day jobs, proving that the path is flexible but demands focus.
For more on how real switchers succeeded, read through student success stories.
How to Navigate the Data Engineering Career Switch
Shifting into data engineering is more than learning a technical skill set — it’s a complete reset on how you use your past experience, manage your time, and showcase new abilities. People from all walks of life, including business managers, nurses, and legacy IT professionals, have made the transition. The right approach breaks this process into manageable steps, lets you measure progress, and helps you stand out in a crowded field.
Evaluating Transferable Skills and Starting Points
Career switchers usually start by looking at what they already know. For example, a business analyst might be adept at solving problems and thinking in terms of processes, while someone from healthcare could be detail-oriented and understand compliance. Legacy IT professionals bring system thinking and problem-solving, although their tech stack may be outdated.
However, most find common skill gaps in:
- Python is essential. Many career changers need to build fluency from scratch.
- While some know the basics, data engineering interviews demand deeper hands-on SQL and performance tuning.
- Platforms like AWS, Azure are now standard for data engineers, but many from non-cloud IT backgrounds need to build this knowledge from the ground up.
It’s normal to realize that keeping up with new technical skills is the single fastest way to close the pay gap — sometimes just a few new tools can turn a business analyst or developer into a strong data engineering candidate. Always assess which skills you have that connect to the technical requirements, and identify clear learning goals to fill the largest gaps.
Creating a Practical, Flexible Study Schedule
Consistency is key during the career transition. Most successful data engineers started with a daily learning commitment. Whether you commit 10 or 60 minutes per day, sticking to a schedule creates powerful momentum. Flexibility is important: some learners blitz through modules in weeks, while others take several months depending on work or family needs.
Here are practical steps for building a study plan:
- Set a minimum daily time goal. Even 10 minutes keeps your momentum.
- Focus on depth, not speed. Learning fast won’t help if you don’t absorb the core concepts.
- Avoid comparing your pace. Each switcher’s path depends on background, job status, and personal obligations.
- Adjust as needed. If life gets busy, scale back but don’t stop altogether.
Building these habits helps manage personal motivation and reduces burnout. The habit of daily focus pays off during hard topics, especially in areas like distributed computing or advanced SQL.
Building an E-Portfolio and Real-World Projects
Employers want proof — not just certificates. A strong e-portfolio filled with impactful, real-world projects helps you stand out in data engineering interviews. Even people without formal computer science backgrounds have landed roles by demonstrating problem-solving and ability to execute projects from end to end.
Effective portfolio projects often include:
- End-to-end ETL pipelines using Python and SQL.
- Cloud deployment scenarios, such as spinning up a data warehouse on AWS or GCP.
- Streaming data applications for real-time analytics or monitoring.
- Automated reporting or dashboard builds, showing data delivery for business.
Real students have built portfolios with public datasets, tackling finance, health, or operations use cases. Projects like these prove skills — and confidence — when it’s time to interview. For more tips on organizing your project work, check out our guide to Data Engineering Projects for Beginners and strategies for How to Build a Data Engineering Portfolio That Gets You Hired.
Demonstrating tangible results often leads directly to interview requests — and ultimately job offers — in data engineering, where proof often beats pedigree.
The Power of Data Engineer Academy Personalized Training
Personalized training does more than teach technical skills. It recognizes that every career changer has a story, a set of skills, and a different level of comfort with tech. This approach changes the way professionals upskill or pivot into data engineering, targeting what matters most for real job outcomes. The Data Engineer Academy model adapts to the individual, not the other way around. This is why people land jobs faster, grow confidence, and often see a direct path to higher salaries.
Custom Learning Paths for Unique Backgrounds
Most programs try to fit learners into fixed curriculums, but that doesn’t work for real people. Data Engineer Academy starts with your actual experience — your background, technical abilities, and personal goals. Instead of a one-size-fits-all approach, the team creates a personalized roadmap.
- Full career switchers, like nurses or finance professionals, get a step-by-step plan that bridges every gap between their old role and the requirements of data engineering.
- Upskillers, such as data analysts, get targeted projects that cover only what’s missing, so they can move into bigger roles quickly.
- IT professionals with legacy skills receive help modernizing by adding cloud, Python, or data pipeline experience, often needed for today’s top roles.
This tailored method speeds up results, reduces wasted effort, and produces job-ready candidates. Success stories show that this works across backgrounds — from zero experience in tech to those already in IT but needing a competitive edge. Recent program data shows job offers often arrive within three to six months of starting, a rate that outpaces general bootcamp averages.
For more on how your location and experience can shape salary potential, review the detailed findings in Data Engineering Salaries by City.
Coaching, Community Access, and Application Support
Personalized training doesn’t stop at a learning plan; it brings full support at every stage. Data Engineer Academy students tap into 24/7 community access on Slack, getting answers from a team of experienced coaches and fellow learners. A private channel means no question goes unanswered.
Weekly 1:1 calls help tackle tough topics or break down project challenges. If life gets busy, coaches adapt the plan and keep you on track. This real-time guidance gives students a sense of momentum and community — two factors that research shows speed up skill mastery and job placement.
During the job hunt, the team even applies for roles on your behalf, saving hundreds of hours. This hands-on help stands out, especially as the average data engineering job attracts over a thousand applicants per listing.
Support extends into your first months on the job. Coaches provide help for technical tasks, culture adaptation, or new tools, reducing the stress of that critical transition period.
If you’re interested in a deeper look at our approach to coaching, the personalized coaching for data engineering careers page breaks down the process step by step.
Unlimited Mentorship and Interview Prep
Unlike standard bootcamps that end after a fixed schedule, Data Engineer Academy’s mentorship is truly unlimited. Students retain access to coaches as long as needed — until a job is landed and well into onboarding at the new company.
- Mock interviews simulate real company processes, building both technical and soft skills needed to stand out.
- Behavioral feedback hones your communication and confidence for high-stakes interviews.
- Resume and project reviews turn portfolio work into strong, marketable proof of skill.
This ongoing mentorship gives career changers the tools to compete with experienced engineers. Graduates consistently report feeling prepared—even for complex technical interviews in big companies or startups.
For practical tips on building a standout profile, review How to Become a Data Engineer in 2025 for actionable strategies grounded in hiring data and real outcomes.
Personalized training has proven effective for job-switchers: recent statistics from Data Engineer Academy show a 100% placement rate for those who stick with the program through completion, with starting salaries averaging $94,000 and experienced hires earning well over $130,000. This combination of adaptive support and practical skill development skips busywork and points you toward your next career success.
Ready to hear more from real people? Check out the Data Engineer Academy reviews for a closer look at student success. Their stories can help you decide if this path fits your goals.
Conclusion
Changing your career to data engineering requires planning, persistence, and real investment. The direct and hidden costs — both financial and emotional — are significant, but the payoff can be life-changing. Personalized training reduces wasted time and makes the learning curve manageable, helping more people cross into this field with confidence.
Salaries in US data engineering continue to rise, with entry-level roles averaging $94,000 and experienced professionals earning above $130,000. Personalized support, unlimited mentorship, and project-driven learning all help speed up job placement — over 90% of focused program graduates land jobs within months.
If you’re ready to take the next step and want expert guidance throughout your journey, explore our Personalized Data Engineering Training. The strongest results come from choosing a path tailored to your background and goals, so you see a real return for your commitment.
Thank you for joining this discussion — if you’re considering this transition, start today and build your future in data engineering with focused support.