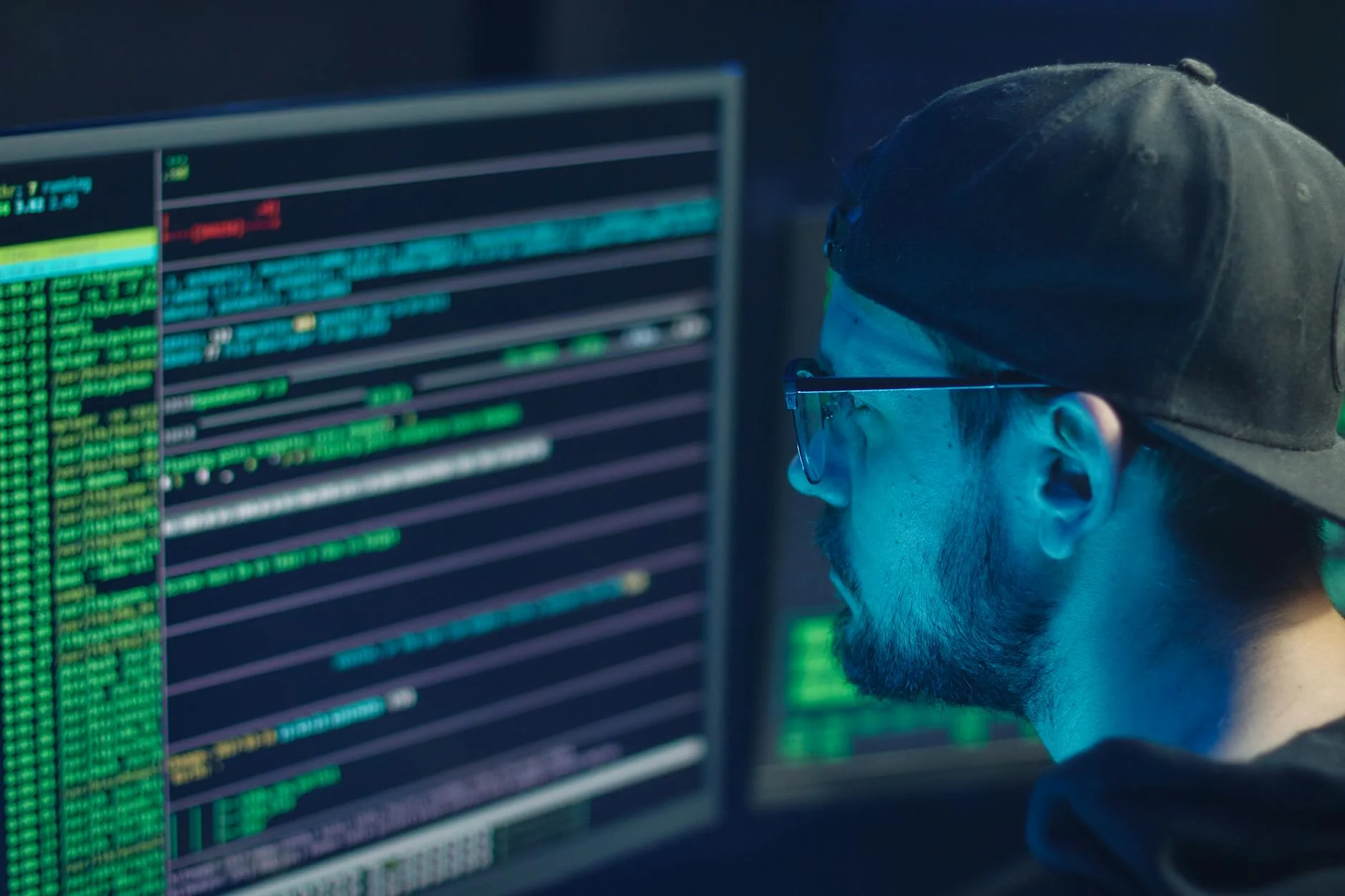
The Rise of the Data Mesh – What You Need to Know
In the fast-evolving world of data management, understanding concepts like data mesh is crucial. So, what’s a data mesh? Simply put, it’s a decentralized approach to data architecture that empowers individual teams to take ownership of their data products. This shift is a response to the growing complexity of data needs within organizations, helping to enhance agility and innovation.
In this post, we’ll dig into the rise of data mesh and explore its significance for data professionals, from system design learners to career changers. You’ll discover how this architecture can drive better data governance and quicker project completion, all while addressing the challenges of traditional centralized models.
Whether you’re navigating your data career or developing new skills, understanding data mesh is essential. So stick around—let’s uncover what you need to know about this transformative approach. For further insights, check out our module on Why Data Engineering is the Fastest-Growing Tech Career.
What is Data Mesh?
Understanding the concept of Data Mesh is vital for anyone stepping into the world of data. It represents a shift in how organizations approach data management—moving away from the traditional, centralized model to a decentralized one. This architecture empowers teams to take ownership of their own data products, ensuring that data is not just stored in silos but leveraged as a shared asset. But what exactly does this entail? Let’s break it down through its core principles.
Core Principles of Data Mesh
Data Mesh is built on four central principles that guide its implementation and functionality:
- Domain-oriented Decentralized Data Ownership: Instead of having a single data team responsible for all data governance, Data Mesh distributes data ownership across different domain teams. Each team manages its own data as a product, meaning they’re responsible for the data quality, privacy, and accessibility within their domain. This approach encourages accountability and can lead to better data quality and more innovative uses of data.
- Data as a Product: In a Data Mesh environment, data is treated as a product rather than a byproduct. Each domain team ensures that their data products are discoverable, understandable, and usable. They focus on the needs of data consumers, providing clear documentation and APIs that facilitate ease of access. This mindset shifts the perspective from merely collecting data to creating value from it. You can delve deeper into the idea of data ownership in this AI-Powered Hiring article.
- Self-serve Data Infrastructure: A strong self-serve infrastructure enables teams to access, manage, and analyze data independently. This element of Data Mesh eliminates bottlenecks that often occur in centralized systems where a limited number of data engineers manage data requests. With self-serve capabilities, domain teams can deploy tools that meet their specific data needs while maintaining security and compliance.
- Federated Computational Governance: Finally, governance in Data Mesh isn’t about strict, top-down controls. Instead, it adopts a federated approach that allows for a balance between local autonomy and global standards. Each domain adheres to guidelines that ensure security, compliance, and quality, while still having the flexibility to innovate within their sphere. This enables a harmonious balance between collaboration and independence across different teams.
By understanding these core principles, you can see how Data Mesh not only optimizes data management but also drives innovation. It’s an empowering strategy that aligns closely with current industry needs, adapting to the pace of change while fostering creativity among data employees.
The Benefits of Adopting Data Mesh
Understanding the benefits of adopting a data mesh is essential for data professionals navigating modern data practices. Transitioning to a data mesh architecture can reshape how your organization handles data, enhances analytics, and fosters collaboration. Let’s explore three significant benefits: increased scalability, improved data quality and ownership, and faster time to insight.
Increased Scalability
Scalability is a top concern for organizations as they grow. Traditional data architectures struggle to keep pace with expanding data volumes and complexity. Enter data mesh, which decentralizes data ownership, allowing different teams to manage their own data. This design facilitates scalability in two key ways:
- Team Autonomy: Each team can independently expand their data products according to needs without waiting for centralized approvals. This leads to faster development cycles as teams can implement and iterate on solutions quickly.
- Adaptive Infrastructure: As businesses evolve, so do their data needs. A data mesh encourages teams to build infrastructure that aligns directly with their goals. They can choose tools and technologies tailored to their specific contexts, whether that’s adapting existing resources or integrating new platforms.
Organizations leveraging data mesh can scale operations seamlessly, continuously adapting to business demands while enhancing analytical capabilities.
Improved Data Quality and Ownership
Decentralization in data management leads to heightened accountability. With data mesh, individual teams take ownership of their data products, resulting in several advantages for data quality:
- Direct Responsibility: Teams that create and manage data also take on the responsibility for its quality. The closer a team is to their data, the more they understand its nuances and imperfections. This fosters an environment where maintaining high data standards is embedded in workflows.
- Tailored Governance: With federated computational governance, each domain sets guidelines that work best for them while staying aligned with organizational standards. This results in a more engaged approach to data governance, enhancing the overall quality of data.
As teams focus on their data, they can better understand the needs of their consumers, leading to significant improvements in both data accuracy and usability. To dive deeper into the role of ownership in data quality, check out this blog on the importance of ownership in data engineering.
Faster Time to Insight
In the fast-paced world of data, delayed insights can hinder decision-making and innovation. Data mesh slashes those bottlenecks, enabling teams to get quicker insights in two critical ways:
- Reduced Dependencies: Centralized models often create slowdowns because teams must wait for data requests to be fulfilled by a single department. In a data mesh framework, teams can access their data directly. This reduces lead times and empowers teams to analyze data on-demand.
- Quicker Experimentation: With self-serve infrastructure, domain teams can experiment with data, running analyses and tests without needing approval from centralized units. This encourages a culture of experimentation and fosters innovation, as teams can pivot based on findings more rapidly.
Faster insights can lead to better decision-making and agility in the business landscape. With a data mesh, organizations can thrive in an environment that demands quick and data-driven decisions. To learn more about efficient data management approaches.
Challenges in Implementing Data Mesh
Navigating the implementation of a data mesh is no small feat. While the advantages are compelling, organizations will inevitably encounter obstacles along the way. Understanding these challenges is crucial for any team looking to transition to a data mesh framework. Let’s break down two significant challenges: the cultural shifts required and the technical barriers that may arise.
Cultural Shift Required
Transitioning to a data mesh isn’t just a technical upgrade; it’s a profound cultural change. Organizations need to rethink their data governance strategies and redefine how teams engage with data. Here’s what that means:
- Empowerment and Ownership: Shifting to a data mesh means decentralizing data ownership. Each team is expected to take responsibility. This empowerment can ruffle feathers if teams aren’t ready for the added accountability. How do you prepare team members for this shift? Start by fostering an environment where ownership is encouraged and recognized.
- Inter-Team Collaboration: In a traditional model, data often flows in a linear path, leading to silos. However, data mesh promotes a more collaborative culture. Teams need to work together, sharing insights and resources. This requires open communication and a rethinking of roles. Are your current workflows set up to manage this change?
- Management Buy-In: For many organizations, leadership may be hesitant to relinquish control over data governance. Gaining their trust is pivotal. Engage them in discussions about the potential for innovation through data mesh. Highlight case studies that show tangible benefits and solutions to common concerns.
Implementing a successful data mesh requires a cultural shift, so companies need a clear strategy for managing these changes.
Technical Barriers and Integration
Integrating a data mesh into existing infrastructures poses its own set of challenges that are tough to navigate. Organizations often find themselves wrestling with a number of technical hurdles:
- Legacy Systems: Most organizations operate on legacy systems that were designed for centralized models. Merging these with a decentralized approach may require extensive re-architecting. How do you bridge the gap? Conduct a thorough assessment of your existing technology stack. Identify which elements can remain and which need updating.
- Standardization of Practices: With data mesh, different teams may adopt varying tools and methods for data management. Without standardization, diversity in practices can lead to confusion and inefficiencies. Creating a guideline can help in maintaining a cohesive framework while still allowing for flexibility.
- Integration Complexity: The technical integration of various data products poses significant challenges. Teams might face difficulties in connecting data pipelines seamlessly. A clear strategy for managing data workflows is essential. Establish a robust data catalog that allows teams to easily discover, access, and use data products, minimizing technical bottlenecks.
Taking the plunge into a data mesh framework means confronting cultural and technical barriers head-on. By addressing these challenges proactively, organizations can set themselves up for success.
Case Studies of Successful Data Mesh Implementations
Exploring real-world examples of data mesh implementations can provide invaluable insights. By examining specific cases, we can better understand not just the hurdles organizations face but also the benefits they realize post-implementation. Let’s take a look at two compelling case studies—Company A and Company B—to see how they navigated their data mesh journeys.
Case Study: Company A
Company A, a well-known e-commerce retailer, faced significant challenges with data fragmentation across various departments. Each team operated independently, leading to redundant data storage and unaligned analytics efforts. They realized that centralizing their data management could not keep pace with their rapidly evolving business needs.
In 2021, Company A transitioned to a data mesh approach, enabling individual teams to take ownership of their data products. The company adopted a “data as a product” mindset. This meant that instead of merely accumulating data, teams had to create datasets that were easily discoverable and usable for others within the organization.
The results? They experienced a 30% reduction in time spent accessing and analyzing data, enabling faster decision-making. Individual teams felt more empowered and responsible for data management, leading to better data quality and more innovative insights. The increased collaboration across departments also fostered a collaborative culture that further enhanced innovation. For a deeper dive into data ownership, consider reading more about data ownership in engineering practices.
Case Study: Company B
Company B, a prominent banking institution, recognized their traditional waterfall methodologies were hindering responsiveness to market changes. They embarked on an ambitious project to transition to a data mesh model that allowed cross-functional teams to manage data as a shared asset.
To kick off their data mesh initiative, they implemented a federated governance model. Each domain was empowered to set its own standards for data quality while adhering to broader corporate guidelines. This balance allowed for flexibility while ensuring that compliance and data security were not compromised.
The benefits were significant: Company B noted a 40% faster turnaround in key analytics reports used for strategic decisions. Stakeholders could now access real-time data, catalyzing more adaptive strategies. In the end, the shift to a data mesh successfully transformed their data culture, producing a more engaged workforce. Interested in learning how organizations have reaped the benefits of data mesh?
These case studies demonstrate how organizations can overcome data challenges and achieve meaningful results by adopting a data mesh approach. They highlight not just the transformative power of decentralization but also the importance of cultural and operational shifts in achieving success.
Future Trends in Data Mesh
As we look ahead, data mesh is poised to play a pivotal role in how organizations manage and utilize their data. With technological advancements and evolving business needs, understanding these future trends will help you better navigate this shifting landscape. Let’s explore how data mesh can seamlessly integrate with emerging technologies and the changing role of data engineers in this framework.
Integration with Emerging Technologies
We’re seeing a rapid growth of technologies like AI and machine learning, and data mesh can take these advancements to the next level. But how does this work?
- AI and Predictive Analytics: Data mesh allows domain teams to directly integrate AI tools into their data products. This means teams can harness predictive analytics to improve decision-making. Imagine your marketing team capitalizing on up-to-date customer data to predict buying behavior and craft targeted campaigns. This level of integration enhances agility and effectiveness.
- Real-Time Data Utilization: Businesses benefit immensely from real-time processing. Data mesh fosters a self-service infrastructure where domain teams can pull real-time data directly into their analyses. This shortens the feedback loop. So, when your sales team needs instant insights for a pitch, they can access the information without bottlenecks from a central data office.
- Collaboration with Automation: Automation is replacing manual processes across various industries. Data mesh can empower teams to embed machine learning models within their data pipelines. This not only streamlines operations but also allows for ongoing learning from the data. It’s like having a built-in assistant that constantly improves and adapts.
By aligning with these emerging technologies, data mesh lays the groundwork for organizations to leverage their data more effectively, turning insights into action. Curious to dive deeper into how organizations are adapting? Check out this overview on the Data Mesh Overview: Architecture & Case Studies for 2025.
The Role of Data Engineers in Data Mesh
As data mesh becomes more prevalent, the roles and responsibilities of data engineers will evolve. It’s crucial to understand how these professionals will adapt within this decentralized framework.
- Transition to Data Product Owners: Instead of solely focusing on data engineering tasks, professionals will need to embrace ownership of their data products. This means becoming advocates for both data quality and usability. Can you envision data engineers collaborating with domain teams to ensure that what they provide is not just reliable but also user-friendly?
- Enhanced Collaboration Across Domains: In a data mesh environment, data engineers will work closely with various domain teams. This collaboration demands strong communication skills and a deeper understanding of domain-specific needs. Breaking down silos will foster a culture where engineers provide tailored solutions that thrive on user feedback.
- Focus on Federated Governance: With a federated governance model, data engineers will be expected to enforce data standards and security measures within their domains. Their role will shift toward monitoring compliance while still encouraging innovation. How do you see this balance affecting your day-to-day? It’s about maintaining integrity while fostering creativity.
In this evolving landscape, data engineers have the opportunity to step into leadership roles that shape the future of data management. Exploring these responsibilities will not only enhance your career prospects but also contribute significantly to your organization’s success in adapting to data mesh. To learn more about trends that are influencing data engineering, take a look at this resource on Future of Data Engineering: Trends for 2025.
Conclusion
The rise of data mesh marks a significant shift in how organizations manage data. By decentralizing ownership and treating data as a product, teams can foster a culture of accountability and collaboration. This approach not only improves data quality but also empowers domain teams to act swiftly, making insights more accessible.
As you consider implementing data mesh in your organization, remember the key principles of autonomy, product ownership, self-service infrastructure, and federated governance. Each principle plays a critical role in enhancing your data practices.
What’s next? Dive deeper into this topic and explore resources on career development in data ownership or check out our Snowflake Integration: Complete Guide.
Thank you for reading, and let’s keep the conversation going—what challenges or thoughts do you have on adopting data mesh?
Real stories of student success
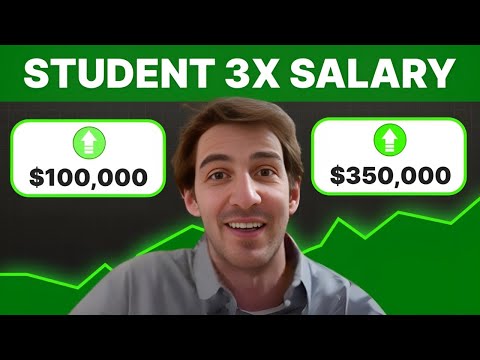
Student TRIPLES Salary with Data Engineer Academy
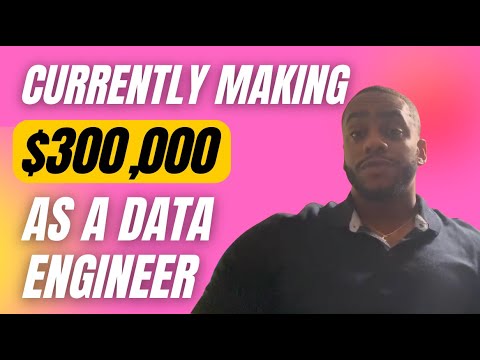
DEA Testimonial – A Client’s Success Story at Data Engineer Academy
Frequently asked questions
Haven’t found what you’re looking for? Contact us at [email protected] — we’re here to help.
What is the Data Engineering Academy?
Data Engineering Academy is created by FAANG data engineers with decades of experience in hiring, managing, and training data engineers at FAANG companies. We know that it can be overwhelming to follow advice from reddit, google, or online certificates, so we’ve condensed everything that you need to learn data engineering while ALSO studying for the DE interview.
What is the curriculum like?
We understand technology is always changing, so learning the fundamentals is the way to go. You will have many interview questions in SQL, Python Algo and Python Dataframes (Pandas). From there, you will also have real life Data modeling and System Design questions. Finally, you will have real world AWS projects where you will get exposure to 30+ tools that are relevant to today’s industry. See here for further details on curriculum
How is DE Academy different from other courses?
DE Academy is not a traditional course, but rather emphasizes practical, hands-on learning experiences. The curriculum of DE Academy is developed in collaboration with industry experts and professionals. We know how to start your data engineering journey while ALSO studying for the job interview. We know it’s best to learn from real world projects that take weeks to complete instead of spending years with masters, certificates, etc.
Do you offer any 1-1 help?
Yes, we provide personal guidance, resume review, negotiation help and much more to go along with your data engineering training to get you to your next goal. If interested, reach out to [email protected]
Does Data Engineering Academy offer certification upon completion?
Yes! But only for our private clients and not for the digital package as our certificate holds value when companies see it on your resume.
What is the best way to learn data engineering?
The best way is to learn from the best data engineering courses while also studying for the data engineer interview.
Is it hard to become a data engineer?
Any transition in life has its challenges, but taking a data engineer online course is easier with the proper guidance from our FAANG coaches.
What are the job prospects for data engineers?
The data engineer job role is growing rapidly, as can be seen by google trends, with an entry level data engineer earning well over the 6-figure mark.
What are some common data engineer interview questions?
SQL and data modeling are the most common, but learning how to ace the SQL portion of the data engineer interview is just as important as learning SQL itself.