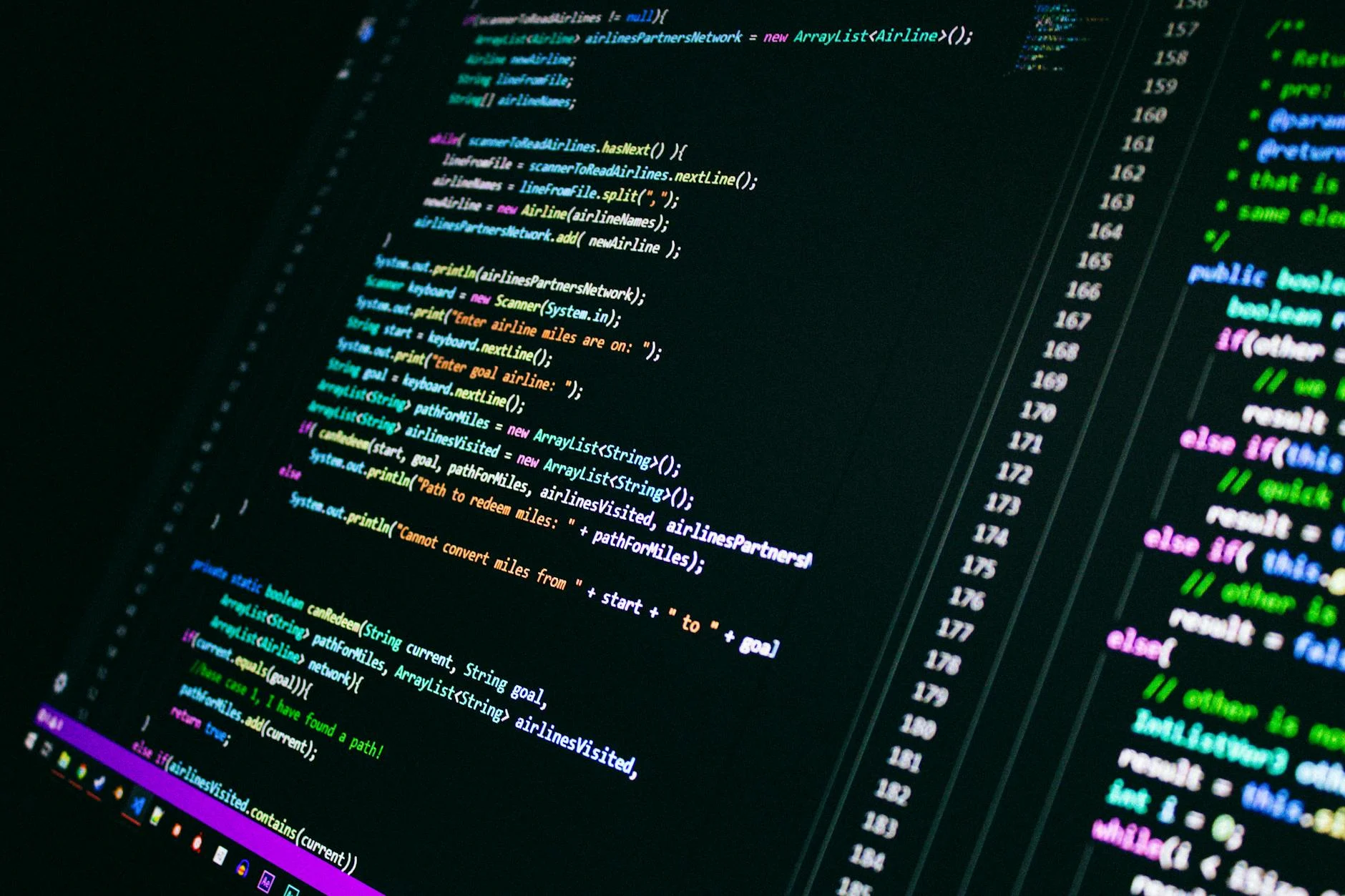
How No-Code and Low-Code Tools Are Changing Data Engineering
The world of data engineering is shifting fast, and you’ll want to pay attention. No-code and low-code tools are at the forefront of this transformation, making data workflows accessible to a broader audience. These platforms let anyone—whether they’re seasoned data engineers or stepping into the field for the first time—build and manage data solutions without deep coding skills.
So, why should you care? These tools streamline the entire process, reducing development time, simplifying data management, and enabling teams to collaborate more effectively. With features like visual programming interfaces and prebuilt connectors, they’re set to change how we think about data engineering.
In this post, we’ll explore how no-code and low-code tools are redefining roles, empowering non-technical users, and enhancing existing frameworks in the data landscape. Whether you’re looking to optimize your skills in data engineering or aiming for a smoother transition into this growing field, you’ll find valuable insights and actionable advice. Let’s jump in and see what these tools can do for you. For more on the benefits of these tools, check out Why Data Engineering is the Fastest-Growing Tech Career.
Understanding No-Code and Low-Code Tools
The rise of no-code and low-code tools is reshaping the data engineering landscape. With their user-friendly interfaces and simplified design processes, these platforms enable a broader range of users— from novice data enthusiasts to seasoned professionals—to engage in data tasks without traditional coding expertise. Let’s break down what these tools are all about.
What is No-Code?
No-code tools are platforms that allow individuals to create applications or workflows without any programming knowledge. They are designed for users who may not have a technical background but possess a desire to build solutions quickly and intuitively. Essentially, no-code tools empower users to bring their ideas to life with little to no technical barriers.
These tools typically feature drag-and-drop interfaces, which simplify the app development process. This means that users can focus on what they want to accomplish rather than how to code it. For instance, platforms like Airtable and Bubble allow users to construct databases and web applications through user-friendly graphical elements.
- Target Audience: Primarily aimed at non-technical users, entrepreneurs, and small business owners, no-code platforms also attract curious learners from diverse fields looking to solve specific problems efficiently.
- Popular Examples:
- Airtable: A flexible database solution that offers a spreadsheet-like interface.
- Webflow: A design tool that allows users to build responsive websites visually.
These platforms particularly shine in prototyping and rapidly deploying applications without the need for extensive coding resources.
What is Low-Code?
Low-code tools, on the other hand, require some level of coding knowledge but significantly reduce the complexity and time needed to develop applications. These platforms still cater to a range of users but include those who have a fundamental understanding of coding concepts. Think of low-code as a bridge that simplifies the coding process; it makes development quicker and more accessible without eliminating the need for technical skills altogether.
The purpose of low-code platforms is to accelerate development cycles by providing pre-built components and templates, which allows developers to focus on unique functionalities. Products like OutSystems and the Microsoft Power Platform exemplify low-code environments, enabling developers to collaborate with other stakeholders while creating applications efficiently.
- Purpose: To streamline the development process while still offering the flexibility of custom coding where required.
- Popular Examples:
- Mendix: This platform supports both developers and business experts to innovate collaboratively.
- Microsoft Power Apps: A robust tool that integrates easily with various Microsoft services, allowing users to create applications rapidly.
Many businesses are adopting low-code tools to maintain agility and respond to ever-changing demands, all while maximizing their existing developer resources.
In summary, no-code and low-code tools offer distinct advantages in today’s fast-paced data engineering environment. As you explore these tools, consider how they can fit into your skill set or existing workflows. For an in-depth look at the specific applications of these tools in data engineering, check out Generative AI in Data Engineering: Key Use Cases & Future Trends.
Impact on Data Engineering Workflows
The emergence of no-code and low-code tools is reshaping how data engineering teams operate. With their ability to simplify complex tasks, these platforms are enhancing the speed and efficiency of data workflows. Let’s explore the specific impacts they have on data engineering.
Accelerating Data Pipeline Development
One of the most significant advantages of no-code and low-code tools is their ability to accelerate data pipeline development. Traditionally, building a data pipeline could take days or even weeks, requiring intricate programming knowledge and extensive testing. However, with these tools, developers can drag and drop components into place, quickly assembling their pipelines without writing code line by line.
Imagine having a toolkit where you can simply pick the pieces you need and fit them together like puzzle pieces. That’s what these platforms enable. They provide pre-built connectors and templates to help you get started quickly, allowing teams to shift their focus from coding to problem-solving. This results in faster iterations and more frequent updates, ensuring that data insights can be accessed when they matter most.
- Key Benefits:
- Reduced Development Time: Speed up the time from idea to deployment.
- Ease of Use: Non-technical users can contribute to data projects without extensive training.
- Faster Prototyping: Quickly create proofs of concept for new data initiatives.
For those interested in how these tools influence performance in real-world scenarios, consider checking out The Impact of AI on Data Engineering, where the integration of AI in workflows is also discussed.
Enhancing Collaboration Between Teams
No-code and low-code tools facilitate better collaboration across teams—bridging the gap between technical and non-technical professionals. In data engineering, communication is key. Traditionally, data engineers would need to work closely with data analysts, business stakeholders, and even marketing teams to gather requirements and develop solutions. Misunderstandings can cause delays and frustration.
With these platforms, stakeholders can easily access the tools and start creating their workflows. Business users can visualize their data requirements, making it easier for engineers to understand what needs to be built. This collaborative environment fosters innovation since non-technical members can share ideas and feedback in real-time.
- Advantages for Collaboration:
- Inclusive Participation: Everyone can contribute to the data conversation.
- Visual Interfaces: Reduce reliance on complex documentation or technical jargon.
- Streamlined Feedback Loops: Less back-and-forth leads to a more efficient development cycle.
If you’re keen to see how collaborative tools can fit into your data engineering workflow, check out Inside the Life of a Data Engineer at Lyft – Daily Workflow.
Reducing Dependency on IT Teams
The ability to utilize no-code and low-code platforms allows non-technical users to handle their data needs independently. Imagine you’re in marketing and you need data insights for a campaign. Traditionally, this would involve submitting a request to the IT or data engineering team, often resulting in delays that could hinder decision-making.
With these tools, you’re empowered to create your own reports and dashboards. You no longer need to wait for a developer to set up your data queries. This independence means that IT teams can focus on more complex tasks, rather than being bogged down with numerous requests for simple data retrieval.
- Benefits to Users:
- Empowerment: Users can take charge of their data needs immediately.
- Efficiency: Reduce ticket backlogs and waiting times for data requests.
- Focus on Strategic Tasks: IT can concentrate on building robust systems instead of repetitive tasks.
As you explore ways to incorporate these tools into your workflow, consider the long-term benefits, including increased efficiency and empowerment. For further insight on data engineering strategies, refer to Databricks for Data Engineers: The Ultimate Step-by-Step Guide.
Lead-Up to Trends in Data Engineering
As no-code and low-code tools continue to disrupt traditional data engineering practices, several critical trends are emerging. Understanding these trends can help you adapt and thrive in this evolving landscape. Let’s explore three key areas shaping the future of data engineering: the rise of citizen data engineers, the integration of generative AI, and predictions for the future landscape.
The Rise of Citizen Data Engineers
Citizen data engineers represent a shift towards democratizing data management. These are typically non-technical individuals who use low-code or no-code platforms to perform data tasks that were once the exclusive domain of skilled engineers. According to discussions surrounding the concept, citizen data engineers are stepping into roles traditionally held by data professionals, primarily because of the accessibility of tools designed for ease of use.
You might be thinking, “Can someone without a strong technical background manage complex data tasks?” The answer is becoming increasingly affirmative. More organizations are fostering this trend as citizen data engineers help bridge gaps in their data workflows. They handle data transformation and integration, often bringing unique perspectives to data challenges. This shift means that professionals from various fields—like marketing or finance—can contribute to data projects without extensive training.
Generative AI Integration
Generative AI is making waves in the low-code and no-code space. By automating complex coding tasks and enhancing user experience, generative AI simplifies the app development process. These tools are being integrated into platforms, allowing users to build sophisticated data solutions more effortlessly.
Imagine a world where you can generate code snippets or troubleshoot issues simply by describing your needs in plain language. Generative AI makes that a reality. With its ability to analyze patterns and generate solutions, it is changing the way you approach data management.
Recent insights reveal how this integration can streamline workflows, improve data accuracy, and foster a more collaborative environment.
The Future Landscape of Data Engineering
What does the future hold for data engineering? The landscape is set for significant transformation. As technologies like AI continue to evolve, we can expect a couple of notable trends to emerge.
- Increased Dependency on Automation: Automation will gain traction, enabling more efficient data processing and management. According to forecasts, tools will automate routine tasks, allowing data engineers to focus on more strategic initiatives.
- Heightened Demand for Data Literacy: As non-technical personnel take on data roles, organizations will prioritize training in data literacy. The need for clear understanding of data’s role in business operations becomes paramount.
By staying ahead of these trends, you position yourself to thrive in the evolving world of data engineering, paving the way for your career as the landscape continues to shift.
Challenges and Considerations
As no-code and low-code tools gain popularity, it’s essential to navigate the challenges and considerations that come with them. Although these platforms simplify many aspects of data engineering, they also present unique hurdles that need addressing. Let’s examine some critical areas of concern, from security to the need for technical knowledge.
Security and Compliance Issues
When using no-code and low-code tools, data security and compliance emerge as pressing concerns. Since these platforms often expose data processes to a broader audience, the risk of unsecured data and compliance violations increases. For instance, data may inadvertently become accessible to unauthorized users, leading to potential breaches. This concern isn’t just theoretical; organizations have reported instances where lax security measures on low-code applications resulted in data leaks and compliance issues.
Given the importance of data governance, businesses must conduct thorough evaluations of these tools. Ask yourself: “Are there robust security protocols in place? How do these platforms handle sensitive information?” It’s vital to ensure that the tools comply with regulations like GDPR or HIPAA, which safeguard user data. Furthermore, keeping software updated and monitoring access rights will help mitigate security risks.
For more insights on data management, consider reviewing Data Engineering Best Practices, which highlights ways to secure your data flows while using various platforms.
Limitations of No-Code and Low-Code Tools
While no-code and low-code platforms offer benefits, they come with limitations that can hinder complex project needs. Here are some notable drawbacks to consider:
- Reduced Customization: With predefined templates and components, you may find it challenging to customize workflows deeply. Some scenarios demand unique solutions that these tools simply cannot accommodate.
- Performance Issues: Applications built on these platforms can experience performance bottlenecks, especially as usage scales. Complex operations may not execute efficiently, leading to slow response times and user frustration.
- Vendor Lock-In: Adopting a no-code or low-code solution often means becoming reliant on a specific vendor’s ecosystem. This can stifle flexibility, as switching platforms may involve significant costs and time
Need for Technical Knowledge
Even with the ease of use that no-code and low-code tools provide, a foundational level of technical knowledge remains beneficial. Here’s why having some technical skills is still a worthwhile asset even for someone utilizing these platforms:
- Troubleshooting: When issues arise, knowing the basics of coding or database management can help identify and resolve problems quickly. If you encounter an error, understanding how the underlying processes work will empower you to troubleshoot effectively.
- Optimizing Workflows: Having a grasp of programming concepts enables you to enhance and customize workflows to better fit your specific needs. You may pivot from the default settings to create something that resonates more deeply with your objectives.
- Collaboration with Developers: If you’re leveraging no-code or low-code tools in a team environment, understanding technical concepts bridges the gap between you and your technical counterparts. Communicating requirements accurately will yield better outcomes for your projects.
In many cases, enhancing your technical skills can amplify the advantages of using no-code and low-code tools. For inspiration on blending technical knowledge with practical skills, visit System Design Interviews for Data Engineers: Questions, which provides insights on developing your abilities within the field.
Conclusion
As we’ve explored, the rise of no-code and low-code tools is pushing the boundaries of what’s possible in data engineering. These platforms are not just altering how tasks are completed—they’re fundamentally changing the way we think about data accessibility and collaboration. Now, let’s talk about how you can take advantage of this shift.
Real stories of student success
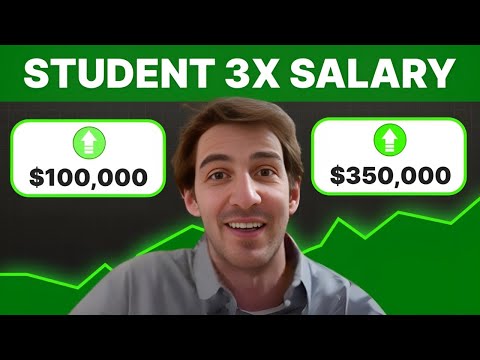
Student TRIPLES Salary with Data Engineer Academy
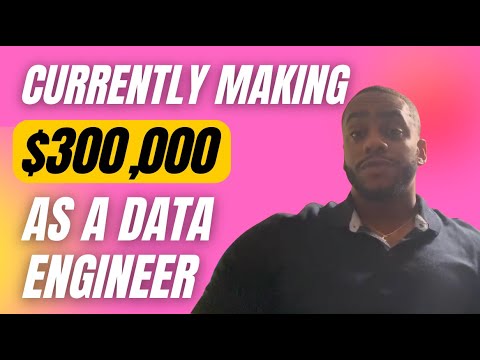
DEA Testimonial – A Client’s Success Story at Data Engineer Academy
Frequently asked questions
Haven’t found what you’re looking for? Contact us at [email protected] — we’re here to help.
What is the Data Engineering Academy?
Data Engineering Academy is created by FAANG data engineers with decades of experience in hiring, managing, and training data engineers at FAANG companies. We know that it can be overwhelming to follow advice from reddit, google, or online certificates, so we’ve condensed everything that you need to learn data engineering while ALSO studying for the DE interview.
What is the curriculum like?
We understand technology is always changing, so learning the fundamentals is the way to go. You will have many interview questions in SQL, Python Algo and Python Dataframes (Pandas). From there, you will also have real life Data modeling and System Design questions. Finally, you will have real world AWS projects where you will get exposure to 30+ tools that are relevant to today’s industry. See here for further details on curriculum
How is DE Academy different from other courses?
DE Academy is not a traditional course, but rather emphasizes practical, hands-on learning experiences. The curriculum of DE Academy is developed in collaboration with industry experts and professionals. We know how to start your data engineering journey while ALSO studying for the job interview. We know it’s best to learn from real world projects that take weeks to complete instead of spending years with masters, certificates, etc.
Do you offer any 1-1 help?
Yes, we provide personal guidance, resume review, negotiation help and much more to go along with your data engineering training to get you to your next goal. If interested, reach out to [email protected]
Does Data Engineering Academy offer certification upon completion?
Yes! But only for our private clients and not for the digital package as our certificate holds value when companies see it on your resume.
What is the best way to learn data engineering?
The best way is to learn from the best data engineering courses while also studying for the data engineer interview.
Is it hard to become a data engineer?
Any transition in life has its challenges, but taking a data engineer online course is easier with the proper guidance from our FAANG coaches.
What are the job prospects for data engineers?
The data engineer job role is growing rapidly, as can be seen by google trends, with an entry level data engineer earning well over the 6-figure mark.
What are some common data engineer interview questions?
SQL and data modeling are the most common, but learning how to ace the SQL portion of the data engineer interview is just as important as learning SQL itself.