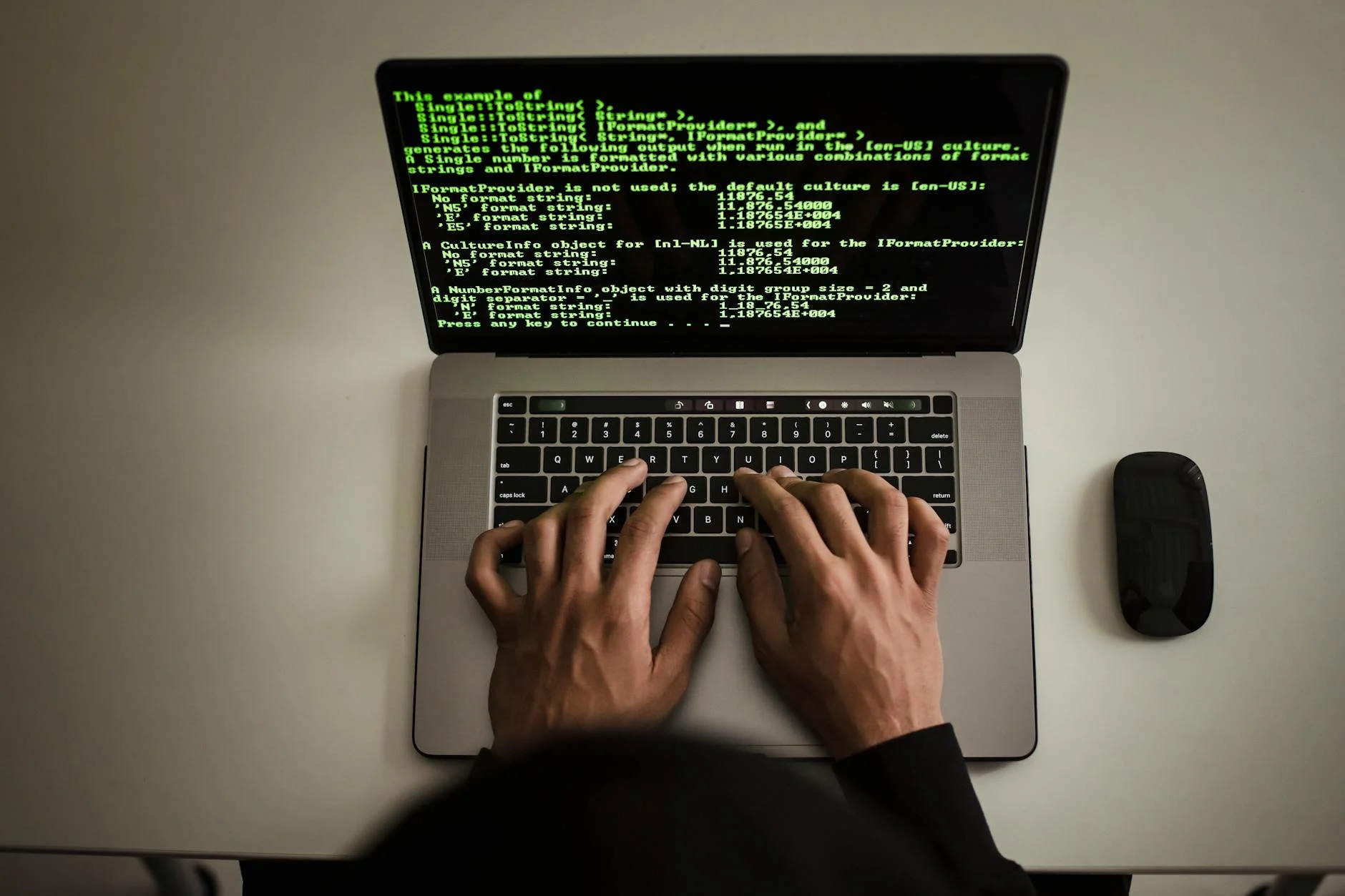
The Importance of Real-Time Data Processing in 2025
Real-time data processing is becoming essential for businesses aiming to thrive in the fast-paced environment of 2025. As we move deeper into this year, the demand for fresh, reliable data continues to grow, especially with the rise of AI-driven applications. This technology enables immediate insights and decisions, fostering efficiency and enhancing customer experiences.
You might be wondering: why is this so critical? Well, real-time data allows businesses to respond swiftly to changing conditions, giving them a competitive edge. With the rise of edge computing, where data processing shifts closer to the source—think IoT devices and smart sensors—latency is significantly reduced, making systems faster and more efficient.
In this blog post, we’ll explore the key trends and technologies shaping real-time data processing, including emerging platforms and tools that facilitate this transformation. Whether you’re a data engineer, system designer, or someone considering a career change in this space, understanding these elements will equip you with insights that are crucial for navigating the evolving data landscape. For those interested in practical applications, resources like 10+ Top Data Pipeline Tools to Streamline Your Data Journey provide valuable guidance on implementation strategies. Join us as we unpack why real-time data processing is not just a trend, but a necessity in today’s business ecosystem.
The Evolution of Real-Time Data Processing
Understanding the evolution of real-time data processing allows us to appreciate its integral role in modern business strategies. With the continuous growth of data generation, it’s not just about collecting information; it’s about how quickly we can use it to drive decisions. Key technologies like edge computing and artificial intelligence are at the forefront of this transformation.
Key Technologies Driving Change
Real-time data processing relies heavily on a few core technologies that shape its landscape. Among these, edge computing and artificial intelligence (AI) stand out.
- Edge Computing: This technology processes data closer to where it is generated, reducing latency and bandwidth usage. Imagine a smart city with sensors collecting urban data; instead of sending everything to a faraway server, edge computing allows for local processing, enabling real-time insights that enhance traffic management, public safety, and environmental monitoring.
- Artificial Intelligence: AI algorithms analyze vast amounts of data quickly, enabling predictive insights and immediate decision-making. Take e-commerce, for example: AI can assess user behavior in real-time to offer personalized product recommendations right when a shopper is about to make a purchase. This capability not only boosts sales but also improves customer engagement.
By integrating these technologies, businesses can harness the power of real-time data processing, allowing for efficiencies that were previously unattainable. Want to explore further? Check out our article on Data Pipeline Design Patterns to understand how data flows in these advanced systems.
Industry Applications of Real-Time Data
Real-time data processing isn’t limited to just one industry; it spans across multiple sectors, each leveraging the technology in unique ways.
- Finance: Financial institutions utilize real-time data to monitor transactions for fraud prevention, manage market fluctuations, and equip traders with timely information that can affect their decisions on investments. By acting instantly, banks can mitigate losses and safeguard client assets.
- Healthcare: In this sector, real-time data processing can be life-saving. Hospitals monitor vital signs through connected devices. Alerts can trigger immediate responses during emergencies, ensuring timely interventions that can significantly impact patient outcomes. Moreover, AI analyzes patient data trends to help predict potential health issues before they escalate.
- E-Commerce: Retailers utilize real-time data to enhance their inventory management and improve user experiences. For example, platforms track consumer behavior actively, allowing businesses to adapt their offerings swiftly, respond to demand changes, and manage supply chains effectively.
In a fast-evolving world, businesses that harness the power of real-time data are better equipped to innovate and meet customer expectations. To get an even deeper insight into how these trends are shaping our futures, consider learning more about the Future of Data Engineering in an AI-Driven World and how it’s transforming industries.
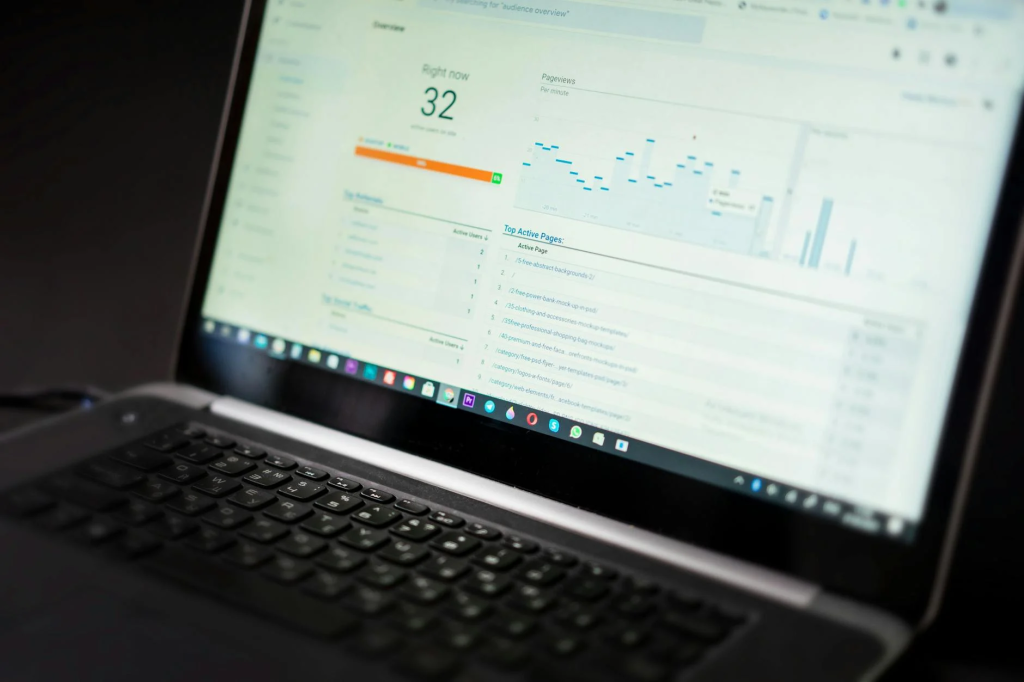
Benefits of Real-Time Data Processing in 2025
As we look toward 2025, the advantages of real-time data processing are more crucial than ever. Businesses that harness the power of immediate data insights can improve decision-making, enhance operational efficiency, and elevate customer experiences. Let’s break down these benefits.
Enhanced Decision-Making
In today’s fast-paced world, the ability to make quick and informed decisions can set a business apart. Real-time data processing offers professionals up-to-the-minute insights, enabling them to respond to market changes and customer behaviors almost instantly. Imagine being able to analyze sales data as it comes in and adjusting your marketing strategy on the fly. This is no longer just a dream—it’s a reality.
- Timeliness: With real-time data, you aren’t relying on outdated reports. Instead, your decisions are grounded in the current state of affairs.
- Accuracy: Near-instantaneous data reduces the chances of errors that might arise from delayed reporting.
- Proactivity: Spotting trends as they emerge allows for proactive steps rather than reactive measures. This can mean the difference between capitalizing on an opportunity and losing it entirely.
Want to deepen your understanding of how real-time analytics can shape decision-making in your field?
Operational Efficiency
Real-time data processing can streamline business processes like never before. Organizations can optimize workflows, manage resources more effectively, and reduce bottlenecks, directly leading to cost savings. Think about it: when information flows smoothly, everything else does too.
- Resource Management: Companies can monitor operations continuously, making adjustments and reallocating resources in real time.
- Workflow Automation: Automated systems can trigger actions—such as inventory restocking or alerting staff to maintenance needs—without human intervention, enhancing productivity.
- Performance Monitoring: Real-time insights help businesses identify which processes are working well and which aren’t, enabling a culture of continuous improvement.
Real-time analytics doesn’t just keep things running smoothly; it lays the groundwork for smarter, data-driven strategies that propel businesses forward. If you’re interested in optimizing your data workflows, consider diving into our guide on Automating ETL with AI for tips on integrating AI into your operations.
Improved Customer Experience
At the heart of any successful business is its customers. Real-time data processing allows organizations to maintain a pulse on customer interactions, preferences, and behaviors, transforming those insights into enhanced experiences.
- Personalization: Real-time insights enable businesses to tailor recommendations and offers that resonate with individual customers. When you receive tailored suggestions based on your browsing history, that’s real-time data working for you.
- Immediate Feedback: Companies can respond to customer inquiries and issues without delay, significantly boosting satisfaction and loyalty. Consider a scenario where a customer encounters a problem; the ability to address it right away matters.
- Optimized marketing initiatives: By analyzing customer engagement as it happens, businesses can adjust campaigns in real time to meet customer needs and maximize impact.
Curious about how this aspect of real-time data impacts various industries? For a broader view, see how revolutionizing customer interactions is not just reserved for one sector but spans across many.
Embracing real-time data processing in 2025 isn’t just about keeping up with trends; it’s about making informed, agile decisions that foster growth and customer satisfaction. By focusing on these key benefits, businesses can position themselves for success in a data-driven future.
Challenges of Implementing Real-Time Data Systems
As the demand for real-time data processing grows, so do the challenges that come with implementing these systems. Businesses eager to capitalize on immediate insights face several hurdles—chief among them are data management and security, as well as integration with existing systems. Let’s break these down.
Data Management and Security
Data governance and security appear at the forefront of concerns when organizations implement real-time data systems. The need for swift access to data can sometimes lead to compromises in how data is managed and protected.
- Governance Issues: In a real-time environment, data is constantly flowing, making it challenging to maintain proper oversight. Organizations need to develop a comprehensive data governance framework that covers data quality, ownership, and compliance with relevant regulations.
- Security Risks: Real-time systems often process sensitive information. This exposure increases vulnerability to external threats—think ransomware attacks and phishing schemes. Cybersecurity measures must evolve to match the agility of real-time data processing, implementing continuous monitoring and adaptive security protocols.
- Data Quality Concerns: Rapid data inflow can sometimes lead to inconsistencies. If data is inaccurate or incomplete, it can compromise the valuable insights that real-time processing aims to deliver. Tools such as automated validation processes can help mitigate these risks.
Integration with Existing Systems
Integrating real-time data processing capabilities with legacy systems can be complicated. Many businesses still rely on established architectures, which sometimes clash with new technologies.
- Compatibility Challenges: Legacy systems may not support the speed and volume of data that modern real-time applications require. Organizations need to evaluate whether to upgrade or completely replace cumbersome systems—a process that can be time-consuming and costly.
- Data Silos: If legacy systems do not effectively share data, businesses may find themselves with fragmented information. This “silo” mentality can thwart the benefits of real-time processing, leaving teams unable to gain holistic insights from their data.
- High Implementation Costs: The transition to a comprehensive real-time data architecture often requires substantial investment. Organizations must allocate resources for training, infrastructure, and possibly new software or platforms.
The journey to implementing real-time data systems can be daunting, but understanding these challenges is the first step toward overcoming them. As organizations seek to refine their data strategies, addressing these areas with targeted solutions will drive success in 2025 and beyond.
Future Trends in Real-Time Data Processing
As we approach 2025, the landscape of real-time data processing is not just evolving; it’s reshaping the way organizations handle data. Understanding these trends will be essential for data engineers and system designers looking to stay ahead. Let’s explore some major shifts you can expect to see.
The Rise of Hybrid Processing Models
In recent years, there’s been a noticeable push towards hybrid processing models that combine both real-time and batch processing. Why does this matter? The answer lies in the unique strengths of both approaches.
- Flexibility: With a hybrid setup, businesses can choose the right processing method for various tasks. For instance, while real-time processing works wonders for immediate analytics, batch processing is still invaluable for complex historical data analysis.
- Improved Efficiency: This model allows for the optimization of resources. You’re able to run intensive batch jobs during off-peak hours without competing with real-time data needs.
- Streamlined Workflows: By merging these processing types, data engineers can create workflows that are both efficient and responsive. This combination can enhance operational agility, enabling businesses to adapt quickly to changing market conditions.
For a deeper dive into how hybrid models function, check out our article on Building Data Pipelines: A Step-by-Step Guide.
Real-Time Data and AI Interdependence
The connection between real-time data processing and artificial intelligence (AI) is becoming increasingly significant. Imagine a situation where real-time data can feed directly into AI systems, essentially creating a feedback loop that enhances both technologies.
- Predictive Analytics: AI can analyze streaming data to predict trends and anomalies instantaneously. This capability is incredibly advantageous for sectors like finance and healthcare, where timely insights can make all the difference.
- Adaptive Learning: By leveraging real-time data, AI algorithms can continuously learn and adapt. This means that over time, they become more accurate and efficient at recognizing patterns, leading to more informed decision-making.
- Enhanced Automation: The combination of these technologies enables more sophisticated automation. For instance, retail businesses can adjust their stocking levels based on real-time sales data analyzed through AI, ensuring they meet customer demand without excess inventory.
Market Forecasts and Opportunities
Looking ahead, the market for real-time data processing technology is projected to expand significantly. The trends indicate opportunities for innovation and investment across various industries.
- Increased Demand for Real-Time Analytics: With businesses increasingly recognizing the importance of immediacy in insights, the demand for tools and technologies that enhance real-time processing capabilities is skyrocketing.
- Growth of IoT Devices: As IoT continues to proliferate, the amount of data generated in real-time will increase dramatically. This trend necessitates more robust real-time data processing solutions to handle the influx.
- Enhanced Focus on Security: As real-time systems become more integral, so does the need for secure processing environments. The importance of cybersecurity measures tailored to real-time data workflows cannot be overstated.
Market analysts are noting not only growth but also the emergence of new business models and tools that leverage real-time insights.
Conclusion
Real-time data processing is not just a trend; it’s a necessity for businesses looking to excel in 2025 and beyond. By harnessing immediate insights, organizations can streamline operations, make quicker decisions, and enhance customer experiences. Technologies like edge computing and AI are key players in this transformation, enabling companies to act swiftly and effectively in a data-driven environment.
As you explore the possibilities, consider how tools like Kafka Streams: Introduction and Databricks for Data Engineers can aid your journey. Whether you’re a data engineer or simply keen on advancing your career, integrating real-time data processing into your strategy will give you a competitive edge. What steps will you take to capitalize on this critical shift in the data landscape?
Real stories of student success
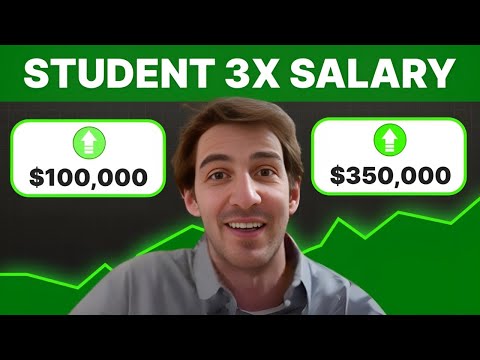
Student TRIPLES Salary with Data Engineer Academy
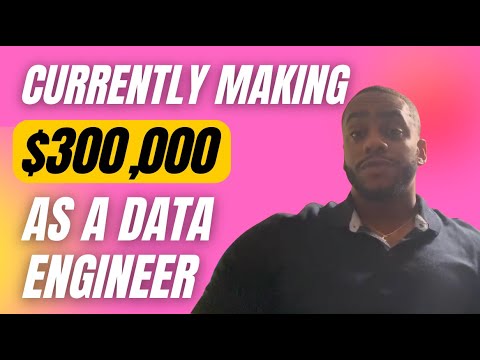
DEA Testimonial – A Client’s Success Story at Data Engineer Academy
Frequently asked questions
Haven’t found what you’re looking for? Contact us at [email protected] — we’re here to help.
What is the Data Engineering Academy?
Data Engineering Academy is created by FAANG data engineers with decades of experience in hiring, managing, and training data engineers at FAANG companies. We know that it can be overwhelming to follow advice from reddit, google, or online certificates, so we’ve condensed everything that you need to learn data engineering while ALSO studying for the DE interview.
What is the curriculum like?
We understand technology is always changing, so learning the fundamentals is the way to go. You will have many interview questions in SQL, Python Algo and Python Dataframes (Pandas). From there, you will also have real life Data modeling and System Design questions. Finally, you will have real world AWS projects where you will get exposure to 30+ tools that are relevant to today’s industry. See here for further details on curriculum
How is DE Academy different from other courses?
DE Academy is not a traditional course, but rather emphasizes practical, hands-on learning experiences. The curriculum of DE Academy is developed in collaboration with industry experts and professionals. We know how to start your data engineering journey while ALSO studying for the job interview. We know it’s best to learn from real world projects that take weeks to complete instead of spending years with masters, certificates, etc.
Do you offer any 1-1 help?
Yes, we provide personal guidance, resume review, negotiation help and much more to go along with your data engineering training to get you to your next goal. If interested, reach out to [email protected]
Does Data Engineering Academy offer certification upon completion?
Yes! But only for our private clients and not for the digital package as our certificate holds value when companies see it on your resume.
What is the best way to learn data engineering?
The best way is to learn from the best data engineering courses while also studying for the data engineer interview.
Is it hard to become a data engineer?
Any transition in life has its challenges, but taking a data engineer online course is easier with the proper guidance from our FAANG coaches.
What are the job prospects for data engineers?
The data engineer job role is growing rapidly, as can be seen by google trends, with an entry level data engineer earning well over the 6-figure mark.
What are some common data engineer interview questions?
SQL and data modeling are the most common, but learning how to ace the SQL portion of the data engineer interview is just as important as learning SQL itself.