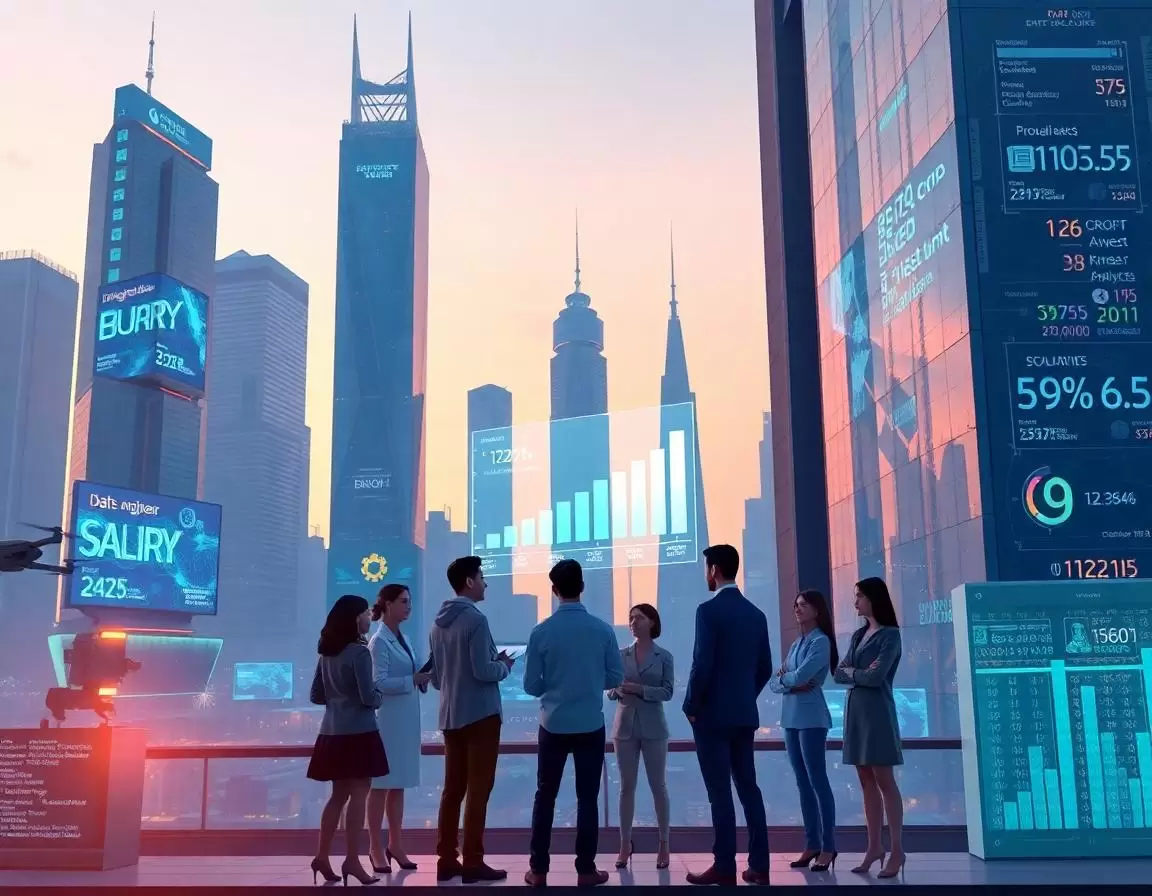
Why Data Engineering is More Lucrative Than Data Science in 2025
Have you noticed how conversations about tech careers always seem to favor data science? Well, 2025 is proving those discussions wrong. Data engineering isn’t just catching up—it’s pulling ahead. Companies are pouring resources into infrastructure over insights, fueling staggering demand for data engineers. Compensation trends? They speak for themselves. Data engineering salaries are growing faster than ever, outpacing data science roles, and the gap keeps widening.
Here’s why: businesses need more robust systems to handle the surge of data before they can even think about analysis. Without the groundwork data engineers lay, those fancy algorithms wouldn’t have a leg to stand on. Personalized training programs, like those offered through Data Engineer Academy, are making it easier than ever to capitalize on this growing demand. And if you’ve been weighing your options, their YouTube channel is packed with resources to get you informed and job-ready.
It’s not just about better pay; it’s a smarter career move—especially if you’re thinking about long-term growth in tech. Here’s what the numbers and trends are saying. Let’s break it down.
The Evolving Landscape of Data Careers in 2025
In 2025, data careers are evolving faster than ever, driven by advancements in technology, shifting business priorities, and a flood of new opportunities. Amid these changes, two roles are standing out: data engineers and data scientists. But here’s the kicker—data engineering isn’t just holding its ground; it’s becoming a standout career choice. Let’s explore the subtleties and trends shaping this landscape.
Data Engineering vs Data Science: Comparing Core Responsibilities
If you’ve ever wondered how these roles differ at a practical level, let’s break it down.
Data engineers are the backbone of any data infrastructure. Their work revolves around:
- Building and maintaining ETL (Extract, Transform, Load) pipelines.
- Designing and deploying automated data pipelines.
- Managing large-scale data storage solutions using tools like Hadoop and Spark.
- Optimizing cloud data platforms, such as AWS, Azure, or Google Cloud.
On the other hand, data scientists focus more on the numbers and outcomes. Their typical responsibilities include:
- Developing predictive models using machine learning.
- Conducting advanced statistical analyses.
- Producing insights from visualizing data and using programming languages like Python and R.
- Creating algorithms to solve business challenges.
Both roles are essential, but the dependency of data science on robust infrastructure is what’s tipping the scales in favor of data engineering. For a deeper dive into the differences between these roles, check out this comprehensive guide on Data Science vs Data Engineering.
Demand Trends in Data Engineering
Here’s a fact: the demand for data engineers is surging. Why? It comes down to scalability. Companies are handling massive datasets, and without experienced professionals to structure, process, and store these assets, even the fanciest AI models fall flat. Demand is particularly intense across sectors like:
- Fintech: Companies revolutionizing payment systems and financial tracking need better data pipelines to ensure accuracy and security at scale.
- Healthcare: The explosion of IoT medical devices and patient records creates a goldmine of data that only qualified engineers can wrangle.
- Retail and E-commerce: With personalized shopping experiences on the rise, robust data infrastructures are critical.
Add to this the rise of cloud platforms like AWS and Azure as the preferred architecture. Knowing your way around them is no longer a bonus—it’s a requirement. That’s why programs like Data Engineer Academy Personalized Training are so valuable in teaching real-world tools and frameworks.
Salary and Growth Disparities Between the Roles
When it comes to paychecks in 2025, the numbers speak for themselves. According to real-time analysis, the projected salaries for data engineers are climbing, driven by demand across industries and geographies.
Here’s a quick comparison:
- Data Engineers:
- Entry-level: $105,000–$130,000.
- Mid-Level: $130,000–$170,000.
- Senior-Level: $170,000–$220,000.
- Data Scientists:
- Entry-Level: $95,000–$130,000.
- Mid-Level: $130,000–$175,000.
- Senior-Level: $175,000–$230,000.
While senior data scientists may edge out slightly in top-end salaries, data engineers are seeing faster salary growth rates year over year. Factors like proficiency in cloud ecosystems, big data technologies, and real-time data streaming are commanding premiums. To catch up with these trends and close any skill gaps, be sure to check out this YouTube channel—it’s a treasure trove of actionable knowledge.
It’s interesting to note that job roles are becoming more specialized, which is also affecting pay. Whether it’s machine learning-focused engineers or big data-specific architects, employers are paying top dollar for tailored expertise.
Why Data Engineering is the Backbone of Modern Tech
Data engineering forms the foundation upon which today’s most innovative technologies are built. It’s not the flashiest tech career, but without it, the apps you use daily, the recommendations you trust, and even groundbreaking AI models wouldn’t be possible. In a world increasingly dependent on data, robust systems and skilled professionals are indispensable. Let’s explore why data engineering is the nerve center of modern innovation.
The Essential Skills for Data Engineers in 2025
In 2025, the core skills for data engineers are evolving alongside emerging technologies. Success in this field demands an updated toolkit and a strong understanding of how to use it effectively. Here’s what every aspiring data engineer needs to master:
- Big Data Frameworks: Platforms like Apache Spark and Hadoop remain crucial for processing massive datasets. Companies rely on these tools to power analytics and real-time decision-making.
- Programming Proficiency: Python and Scala are staples, and SQL is non-negotiable. These languages enable engineers to build pipelines, automate tasks, and manipulate data effortlessly.
- Cloud Expertise: As AWS, Azure, and Google Cloud lead data infrastructure, cloud proficiency is a must-have. If you’re not familiar with cloud platforms yet, make this your next priority.
- Stream Processing: Tools like Apache Kafka are pivotal for real-time data workflows, enabling faster insights and enhancing user experiences.
- Data Storage Solutions: Whether it’s traditional relational databases or NoSQL systems like MongoDB, understanding data storage technologies is foundational.
For a quick breakdown of these skills and a roadmap to mastering them, check out the Key Concepts and Career Roadmap in 15 Minutes. It’s an excellent resource to ensure your knowledge base is up-to-date and industry-relevant.
Examples of Real-World Impact: Data Engineering Insights
The true power of data engineering lies in its ability to transform industries. Let’s look at a few examples that showcase just how impactful skilled data engineering teams can be:
- E-Commerce Giants: Think about personalized shopping experiences. Platforms like Amazon fine-tune their recommendations using pipelines built and maintained by data engineers. Their systems process petabytes of behavioral data in real time, ensuring every product suggestion feels oddly perfect.
- Distributed Systems in Streaming: Platforms like Netflix optimize bandwidth and deliver buffer-free viewing globally. How? By leveraging data engineers to create infrastructure that constantly analyzes server load and user behavior to adapt streaming quality.
- AI-Powered Healthcare: From predictive diagnostics to tailored patient care, healthcare companies are investing heavily in data pipelines. These systems organize and preprocess enormous datasets so machine learning models can identify patterns and save lives.
- Transportation Networks: Ridesharing companies like Uber use data engineering to track millions of rides per day, optimize routes, and dynamically adjust pricing based on demand.
This kind of success doesn’t just happen—it’s built on the shoulders of skilled data engineers. Want to explore why this career is exploding in demand? Check out Why Data Engineering is the Fastest-Growing Tech Career, which dives deeper into the role’s critical importance.
If becoming a part of this movement sounds like your next step, the Data Engineer Academy’s Personalized Training can help you master these technologies with hands-on, real-world guidance. Their YouTube channel is also a goldmine of free tutorials to get you started.
This isn’t just about building your career; it’s about driving innovation and being at the center of technology’s future.
Career Development and Upskilling Opportunities
The landscape of tech careers is constantly shifting, and staying relevant means keeping up with evolving skills and tools. For data engineers—the backbone of modern data systems—there’s a wealth of resources available to sharpen your expertise, change career paths, or simply boost your earning potential. Let’s dive into the strategies that can help you achieve your goals.
Online Learning Platforms vs Hands-on Training
If you’re looking to enhance your skills or transition into data engineering, education is your first step. But what’s the best approach—self-paced online courses or immersive, hands-on programs? Both have their merits, but the answer depends on your learning style and career timeline.
Online Learning Platforms
Traditional platforms like Coursera or Udemy offer flexibility. You can study Python fundamentals, SQL commands, or data pipeline basics at your own pace, often at an affordable price. These platforms are great for foundational knowledge and offer access to a variety of introductory material. However, they often lack depth when it comes to practical, job-specific scenarios.
Hands-on Training Programs
For career shifters aiming to immediately stand out in job applications, immersive programs like the Data Engineer Academy Personalized Training provide a real edge. Such programs focus on project-based learning, where you get to deploy pipelines, work with live data, and build solutions reflecting current industry challenges. This type of practical experience isn’t just about understanding concepts—it’s about applying them with confidence.
Quick Comparison:
- Online Platforms Pros: Budget-friendly; great for beginners; self-paced.
- Online Platforms Cons: Lacks depth; minimal real-world application.
- Hands-on Training Pros: Immersive; job-focused; includes mentorship and guidance.
- Hands-on Training Cons: Costs can be steeper, and it typically requires a more fixed time commitment.
Certifications to Elevate Your Resume
Certifications are a direct pathway to showcasing your skills and signaling to employers that you’re qualified and ready for the job. But which certifications hold the most weight for data engineers looking to thrive in 2025?
Here’s a list of certifications worth pursuing:
- AWS Certified Data Analytics Specialty: Demonstrates expertise in building and managing AWS data analytics solutions, a must-have for cloud-based roles.
- Microsoft Certified: Azure Data Engineer Associate: Covers designing and implementing data pipelines on Azure, a critical skill given Azure’s growing market presence.
- Google Professional Data Engineer: A high-value credential if you want to work on GCP, addressing tasks like merging and transforming raw data into actionable insights.
- Databricks Certified Data Engineer Associate: Ideal for harnessing large-scale data processing platforms like Apache Spark.
To know the differences in platform demand, you can also explore insights from AWS vs Azure data engineering: Which is more in demand?. It’s a fantastic resource for understanding where to focus your certification efforts.
Certifications don’t just look good on paper; they build real skills that you’ll often use in workplace settings or interviews. Plus, they show a level of dedication that sets candidates apart, especially in competitive hiring markets.
Community Engagement and Networking
Here’s a truth that’s easy to overlook: learning doesn’t end with certifications or skills. Building relationships within professional communities can open doors to hidden job opportunities, collaborations, and even mentorship.
Where to Start?
- Online Forums and Meetups: Platforms like LinkedIn Groups or Reddit tech communities are packed with professionals sharing advice, job leads, or hiring trends.
- Educational Video Resources: Join the thriving community at Data Engineer Academy’s YouTube Channel. From practical tutorials to career tips, their videos are designed to upskill while keeping you engaged.
- Local and Virtual Events: Attending tech meetups or virtual conferences builds connections with people who could be your next employer, mentor, or collaborator.
One standout example comes from the ethos shared by those who’ve successfully transitioned careers, as highlighted in this Student Success Story. Networking within such programs often creates a ripple effect of opportunities—proven by careers that see salary leaps or enviable promotions.
The beauty of building community ties is not just about landing jobs—it’s about staying connected to the industry’s pulse. You’ll know which tools to adopt, which skills to refine, and even which companies are embracing cutting-edge methodologies before anyone else.
Future Outlook: Why Data Engineering Will Continue to Shine
When you think about careers with staying power in tech, data engineering unquestionably makes the list. Companies across industries—from media to healthcare to fintech—are doubling down on building richer and more scalable data systems. But what sets data engineering apart isn’t just its current importance—it’s the trajectory. The skills and technologies within this field align with where businesses are headed, making it one of the most future-proof roles in tech. Let’s break down why.
Emerging Technologies Shaping the Field
The rate of innovation in data engineering isn’t slowing down. New tools and paradigms are shaping how data is processed, stored, and delivered in real time. Let’s highlight the game-changers:
- Machine Learning Operations (MLOps): Data engineers are increasingly tasked with creating the infrastructure needed for ML pipelines. This means combining datasets, preprocessing data, and ensuring the scalability of models. As machine learning gets more operationalized, data engineers are essential in turning it into a predictable, scalable workflow.
- Real-Time Data Streaming: Platforms like Apache Kafka and Amazon Kinesis are becoming standard. Companies need to process large streams of data instantly—think of fraud detection models at banks or live-tracking services like Uber. Real-time data streaming is no longer a luxury; it’s a necessity.
- AI-Driven Data Platforms: Automation is entering even the data engineering world, but rather than making jobs obsolete, it enhances productivity. AI tools are automating repetitive ETL (Extract, Transform, Load) tasks, giving engineers more room to solve high-level challenges. Tools like DeepCode AI are already paving the way for accelerated yet precise engineering.
These trends show that the skillset of a data engineer will only continue to expand. Staying informed is critical, which is why resources like Data Engineer Academy Personalized Training are so valuable—they focus on both foundational skills and emerging technologies at the forefront of the field.
Long-Term Career Sustainability
If job security is key to a great career, then data engineering is the jackpot. Why? It’s a foundational role that adapts effortlessly to different tech trends. Here’s how it stands out for long-term sustainability:
- Building Core Skills for Broader Roles: Data engineers often transition seamlessly into positions like data architects, cloud engineers, or machine learning specialists. The foundational knowledge of pipelines, databases, and cloud environments gives them an edge in adapting to new roles. Compare this to specialized fields where pivoting might require starting from scratch.
- Resilience to Automation: While some tech roles face automation threats, data engineering thrives. Why? Because every new automation tool first requires robust infrastructure—something only experienced data engineers can build and maintain.
- Growing Demand Across Pioneering Industries:
- Healthcare: With health tech companies implementing advanced analytics and predictive healthcare tools, the demand for robust, scalable data handling grows.
- E-commerce: As more platforms offer personalized shopping and real-time tracking systems, data engineers are at the heart of it all.
- Green Technology: The rise of sustainability-focused businesses means new, rich data streams that need managing, from climate data to energy usage.
Investing in data engineering isn’t just a smart move for now—it’s a future-proof one. To learn how this career can evolve with your skills, it’s worth exploring resources like How to Build a $300K Career as a Data Engineer for clear, actionable insights.
Even if you’re already in tech, there’s always room for growth. Check out the Data Engineer Academy’s YouTube channel to stay ahead of the curve with free tutorials and advice from industry leaders. This is one field where shot callers are made, not replaced.
Conclusion
Data engineering’s rising prominence in 2025 is no coincidence—it’s a result of businesses prioritizing scalable, robust infrastructure to handle the vast amounts of data they generate daily. This focus on creating systems that enable efficiency and innovation makes data engineering not just a lucrative career but a smart, future-proof investment.
For those considering the shift or looking to upskill, there’s no better time to dive into this field. Practical resources like the Data Engineer Academy Personalized Training offer hands-on projects to bridge the gap between knowledge and application. And if you’re just exploring, their YouTube channel is packed with tutorials and insights to get you started.
So, here’s the big question: Are you ready to future-proof your career in tech? The opportunities are waiting—but only for those who take action.
Real stories of student success
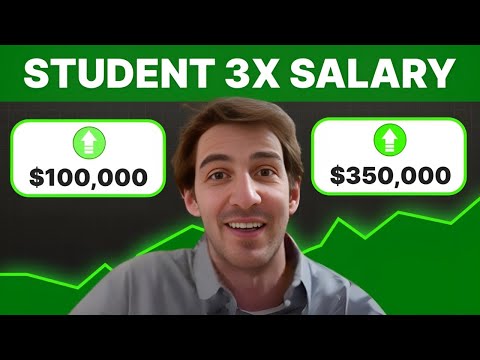
Student TRIPLES Salary with Data Engineer Academy
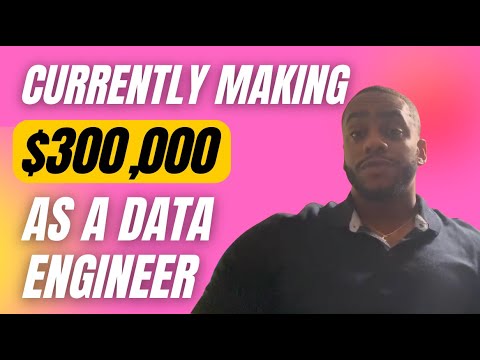
DEA Testimonial – A Client’s Success Story at Data Engineer Academy
Frequently asked questions
Haven’t found what you’re looking for? Contact us at [email protected] — we’re here to help.
What is the Data Engineering Academy?
Data Engineering Academy is created by FAANG data engineers with decades of experience in hiring, managing, and training data engineers at FAANG companies. We know that it can be overwhelming to follow advice from reddit, google, or online certificates, so we’ve condensed everything that you need to learn data engineering while ALSO studying for the DE interview.
What is the curriculum like?
We understand technology is always changing, so learning the fundamentals is the way to go. You will have many interview questions in SQL, Python Algo and Python Dataframes (Pandas). From there, you will also have real life Data modeling and System Design questions. Finally, you will have real world AWS projects where you will get exposure to 30+ tools that are relevant to today’s industry. See here for further details on curriculum
How is DE Academy different from other courses?
DE Academy is not a traditional course, but rather emphasizes practical, hands-on learning experiences. The curriculum of DE Academy is developed in collaboration with industry experts and professionals. We know how to start your data engineering journey while ALSO studying for the job interview. We know it’s best to learn from real world projects that take weeks to complete instead of spending years with masters, certificates, etc.
Do you offer any 1-1 help?
Yes, we provide personal guidance, resume review, negotiation help and much more to go along with your data engineering training to get you to your next goal. If interested, reach out to [email protected]
Does Data Engineering Academy offer certification upon completion?
Yes! But only for our private clients and not for the digital package as our certificate holds value when companies see it on your resume.
What is the best way to learn data engineering?
The best way is to learn from the best data engineering courses while also studying for the data engineer interview.
Is it hard to become a data engineer?
Any transition in life has its challenges, but taking a data engineer online course is easier with the proper guidance from our FAANG coaches.
What are the job prospects for data engineers?
The data engineer job role is growing rapidly, as can be seen by google trends, with an entry level data engineer earning well over the 6-figure mark.
What are some common data engineer interview questions?
SQL and data modeling are the most common, but learning how to ace the SQL portion of the data engineer interview is just as important as learning SQL itself.